Five Predictive Imperatives for Maximising Customer Value
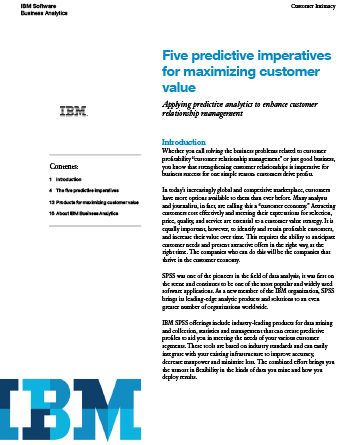
In today's increasingly global and competitive marketplace, customers have more options available to them than ever before. Many analysts and journalists, in fact, are calling this a "customer economy." Attracting customers cost effectively and meeting their expectations for selection, price, quality, and service are essential to a customer value strategy. Download this whitepaper to learn the 5 best practices used by leading organisations to maximise customer value with predictive analytics.
Get the downloadBelow is an excerpt of "Five Predictive Imperatives for Maximising Customer Value". To get your free download, and unlimited access to the whole of bizibl.com, simply log in or join free. |
![]() |
Five predictive imperatives for maximizing customer value
Applying predictive analytics to enhance customer relationship management
Introduction
Whether you call solving the business problems related to customer profitability “customer relationship management” or just good business, you know that strengthening customer relationships is imperative for business success for one simple reason: customers drive profits.
In today’s increasingly global and competitive marketplace, customers have more options available to them than ever before. Many analysts and journalists, in fact, are calling this a “customer economy.” Attracting customers cost effectively and meeting their expectations for selection, price, quality, and service are essential to a customer value strategy. It is equally important, however, to identify and retain profitable customers, and increase their value over time. This requires the ability to anticipate customer needs and present attractive offers in the right way, at the right time. The companies who can do this will be the companies that thrive in the customer economy.
SPSS was one of the pioneers in the field of data analysis; it was first on the scene and continues to be one of the most popular and widely used software applications. As a new member of the IBM organization, SPSS brings its leading-edge analytic products and solutions to an even greater number of organizations worldwide.
IBM SPSS offerings include industry-leading products for data mining and collection, statistics and management that can create predictive profiles to aid you in meeting the needs of your various customer segments. These tools are based on industry standards and can easily integrate with your existing infrastructure to improve accuracy, decrease manpower and minimize loss. The combined effort brings you the utmost in flexibility in the kinds of data you mine and how you deploy results.
Many CRM initiatives fail to generate expected returns
Businesses in virtually every industry have implemented CRM strategies. Some have been massive initiatives, supported by significant investments in technology and designed to shift a company’s orientation from products to customers. Many of these initiatives, however, are failing to generate the expected returns and deliver significant value. This is partly due to the difficulty of pushing change through established cultures or processes. But another factor is that although operational CRM systems such as sales force automation or call center systems provide the necessary foundation for better customer relationships, they don’t do much to improve organizations’ ability to maximize customer lifetime value.
The lifetime value of a customer is defined as the total profits generated during the time the customer does business with your company. The concept behind maximizing customer lifetime value is simple: Deliver value to customers cost effectively. When your organization delivers what customers need – what’s valuable to them – customers are more likely to remain open to future marketing efforts, buy more of your products and services and, as a result, become more valuable. This is a win-win relationship for both you and your customer. However, to achieve and maintain this type of relationship requires support from both operational and analytical CRM systems.
Analytics drives CRM returns
Until recently, analytics didn’t come up often in CRM conversations. Now, though, leading CRM experts are nearly unanimous, noting that analytics not only improves CRM efforts, but is essential to their success.
Companies typically start with historical analytics, using a combination of reporting tools, specialized data warehouses, and online analytical processing (OLAP) solutions. As mentioned above, these solutions focus on understanding and measuring the outcome of past decisions and results, and can be useful in narrowing the scope of further investigations. But on their own, they can’t provide your organization with a clear picture of the future.
Industry leaders, however, are evolving their analytical capabilities by adding data mining and other predictive capabilities to their operational CRM systems. Data mining is the process of discovering meaningful and previously unknown correlations, patterns, and trends in large amounts of data. To make these discoveries, data mining relies on pattern recognition technologies, and statistical and mathematical techniques. Because it is forward-looking, data mining enables your organization to measure the potential of your customer relationships and develop plans to maximize that potential.
The most evolved analytical CRM solutions continuously apply predictive analytics technologies and deploy the results enterprise-wide, so that whether customers interact with your organization online, by phone, or face-to-face, they receive the kind of treatment that meets their present needs and anticipates new ones. This increases their tendency to remain loyal and make additional purchases, increasing their lifetime value – and your organization’s profits.
For example, one of the world’s largest airlines relies on predictive analytics to optimize revenue for each flight, improve service for its best customers and increase customer loyalty. By using IBM SPSS Modeler for predictive modeling, its agents and flight staff were able to identify high-value customers and ensure that their needs were met. The resulting increase in customer satisfaction boosted annual revenues – an average of $200 each for its “valuable” customers and $800 each for its “most profitable” customers. Total revenues for the year after implementation increased by $40 million. Over the same period, the airline saved $31 million in operational costs.
The five predictive imperatives
Based on extensive experience with a wide range of organizations, our company has identified the following “predictive imperatives” – best practices used by leading organizations to maximize customer value with predictive analytics.
- Base your customer strategy on predictive profiles
- Predict the best way to win the right customers
- Predict the best way to grow customer relationships
- Predict the best way to keep the right customers longer
- Use predictive intelligence at every customer touch-point
1: Base your customer strategy on predictive profiles
Detailed, accurate predictive profiles are the essential foundation of any customer strategy and CRM initiative. To understand your customers better, use analytical tools to create customer segments, and then create predictive profiles of each segment. These profiles, when deployed enterprise-wide, enable your entire organization to focus on activities that are most likely to generate the highest returns.
Identify key customer segments
You can define customer segments based on behavioral information drawn from operational systems and on attitudinal information obtained through market research. The two approaches complement each other, enabling you to gain a more accurate customer understanding and develop more effective strategies for each customer segment.
You can segment customers and prospective customers according to a number of different criteria. For example, you can analyze customers by the amount they spend with you, by their payment pattern, by the length of the relationship, and many other factors. You can split customer segments into smaller sub-segments, even reaching the ultimate one-to-one relationship, in which you understand each individual’s needs and preferences. By understanding which customers are most likely to purchase certain products or services, you can focus your marketing programs to obtain the highest possible response on your marketing investment. You can segment customers by value, behavior, demographics and even by attitude.
- Segmentation by value builds an understanding of who your most valuable customers are
- Segmentation by behavior helps you know who is most likely to purchase your products or services, so you can use marketing funds more effectively
- Segmentation by demographic and other supplemental data provides additional information that can be used in predicting customer behavior
- Segmentation by attitude adds another dimension to your customer understanding. One of the best ways to understand customers’ attitudes is to ask them through survey research.
Create predictive profiles of each segment
Once you’ve identified the segments of customers who use and value your products and services, the next step is to understand what products or services customers in each segment are likely to want next. Adding this predictive element makes your customer relationship significantly more productive and profitable.
One of the world’s leading financial services companies initiated a customer loyalty program using IBM SPSS Modeler. The data mining solution enabled the firm to analyze its data warehouse of 2.5 million customers according to 400 different attributes. By defining a number of different customer segments, the company could focus its marketing campaigns on the one percent who are not only “extremely likely” to purchase a product or service, but also have the credit rating to do so. It recouped its investment in the project within two years.
2: Predict the best way to win the right customers
Acquiring customers is costly but necessary. Paying too high a price to attract customers, however, or acquiring the wrong types of customers, can have a significant negative impact on your profits.
Using inefficient methods to attract customers will result in higher costs and profits that are lower than they should be. Attracting the wrong customers impacts profits, too. For example, if you attract customers who are likely to leave or “churn,” you may incur the acquisition cost without ever seeing a profit from the customer relationship. Other customers may be loyal, but cost so much to serve that they are only marginally profitable.
IBM SPSS predictive analytics minimizes your organization’s costs by directing programs toward the people most likely to respond. You can further boost profits by focusing on the types of prospects most likely to become profitable customers.
Create a prediction-based customer attraction strategy
Use predictive profiles to determine what types of customers you want to attract. Then create a cost-effective attraction strategy that includes separate plans for each customer segment.
Most companies will want to focus their attraction efforts on winning over prospects that fit the profile of their most profitable customers. But other, less-profitable customer segments may have more room to grow over the long term, or may be more cost-effective to attract – so marketing to these segments may be an attractive option when marketing budgets are tight.
Optimize your customer attraction strategy with response modeling
Fine-tune your customer attraction plans by using response modeling to predict which marketing programs will generate the highest response. This benefits your organization in two ways: you attain the results you want, while avoiding the high costs associated with unproductive marketing efforts. In this way, you see higher profits for the money you invest.
A large Belgium-based insurance company saw its profit margin narrowed and its growth strategy threatened because the cost of adding new customers exceeded revenues from first-year premiums by almost 50 percent. IBM SPSS predictive analytics and decision management technologies allowed the firm to first identify groups most likely to respond to a campaign, and then perform a sophisticated profit-cost analysis. Using this information the company reduced its direct marketing costs by 30 percent and made acquisition campaigns profitable in the first year. In addition, long-term customer profitability increased by 20 percent.
Improve conversion rates with prospect surveys
Market research can be used to improve customer acquisition both before and after your campaigns. Beforehand, surveys of groups identified as likely prospects can clarify their reasons for buying your products or services, enabling you to refine your campaign offers. Afterwards, by surveying prospects that did convert and those that did not, you can learn what worked, and what you need to change, to earn prospects’ business in the future. By using this type of predictive intelligence to guide your customer attraction strategy, you can improve the conversion rate for your best prospects.
3: Predict the best way to grow customer relationships
To maximize customer growth and increase customer lifetime value, your organization needs to know not only what customers are most likely to want, but also when and how they will want it delivered. With IBM SPSS predictive analytics, you can achieve this level of customer knowledge.
Create a prediction-based customer growth strategy
By using predictive profiles, product-affinity models, segmentmigration models, response models, and even survey research, you can generate predictive intelligence about your customers. As a result, your customers will be more satisfied with your service, and reinforce their decision to buy from you again.
Discover product affinities
Customers often purchase products and services together, or in certain sequences. By analyzing their “market baskets” – products and services purchased at the same time – you can offer customers appropriate additional products at just the right time. Understanding what products your customers buy together can lead to improved product placement in retail stores, attractive “bundling” of products in both direct marketing and online offers, and more timely offers. Not only does this increase revenues, it generally improves customer satisfaction and contributes to maximizing customer lifetime value.
A leading computer retailer in Japan, used IBM SPSS Modeler to build a recommendation engine that suggests products to visitors to its website. Recommendations are based on customer profiles and information about prior purchases contained in the company’s database. The first year it was implemented, the recommendation engine resulted in a sales increase of 18 percent, and a profit increase of 200 percent.
Predict segment migration
Applying data mining techniques to your historical sales data shows you who buys what. By combining this information with other data, you can also make other kinds of predictions, such as which customer segments will become more valuable and which less valuable, and by what amount. Predictive segmentation modeling shows you which characteristics are linked to migration between customer value segments. Adding this kind of predictive intelligence to your customer growth strategy enables you to realistically plan growth for each segment.
Optimize your customer growth strategy with response modeling
Fine-tune your customer growth plans by using response modeling to predict which types of marketing programs will generate the highest response. A firm that operates 15 vacation parks initiated a growth strategy that included improving its occupancy rate and cross-selling more sports and leisure activities to guests. Using IBM SPSS predictive analytics and decision management technologies, the company added smaller, more targeted mailings to its marketing strategy. This reduced direct marketing costs by close to $1.5 million in a single year, while increasing revenues by $1.65 million.
Grow relationships by asking customers what they want
Using the data you already have to predict customer needs is a powerful way to improve interactions and lifetime value. But it is also important to systematically ask customers what they want. Surveying your customers and gaining a better understanding of their needs, and why they buy from you, enables your organization to improve your customer growth strategy and maximize customer lifetime value.
4: Predict the best way to keep the right customers longer
Studies have shown that customer acquisition can cost five to 12 times more than retention, and that improving its customer retention rate by just five percent can increase an organization’s profitability by from 25 to 100 percent. Obviously, improving customer retention can have a big impact on profits.
Customer attrition is particularly challenging for online retailers and companies in financial services, telecommunications, and other industries where customers can change vendors relatively easily.
Create a prediction-based customer retention strategy
Keep your best customers longer by creating attrition models, and then use these models to determine which customers are at risk of defecting. You can enrich these models through survey research that adds valuable attitudinal information.
Create predictive attrition models
Understand which customers are most likely to leave for competitors and, more importantly, why. By applying data mining techniques to data about your customers, you can develop profiles of customers who are valuable and customers who have previously defected. Then you can develop strategies to keep your valuable customers from leaving.
A multinational European bank with more than one million customers successfully reduced customer attrition with data mining. IBM SPSS Modeler helped it identify the key behaviors of customers likely to leave the bank, so it could do what was needed to keep them. By focusing retention efforts on its most valuable customers, the bank reduced attrition by 15 to 20 percent and increased profits by 10 to 20 percent.
A European telecommunications company also uses IBM SPSS Modeler to identify customers likely to leave or “churn.” By discovering what types of customers were likely to leave, the company was able to make targeted offers that reduced churn by 20 percent, compared to a similar group that did not receive the offer.
A U.S.-based telecommunications company used IBM SPSS text analytics and data mining technologies to predict and prevent churn. It increased the effectiveness of its attrition model by 10 percent, saving hundreds of thousands of dollars and putting itself in a stronger competitive position in its industry.
Conduct and analyze satisfaction surveys
Satisfaction surveys are invaluable in determining not just whether customers are satisfied, but why, and in uncovering issues that may affect their future loyalty in time to take corrective action. Even customers that you aren’t able to retain have potential value to your organization. By surveying customers that you failed to retain, you can better understand what you need to do to keep customers like them.
A Netherlands hospital that serves nearly 120,000 patients annually needed to comply with the requirements of national healthcare quality legislation. In addition to monitoring mandated quality standards, the hospital also wanted to monitor patients’ opinions and preferences. The hospital chose the IBM® SPSS® Data Collection survey research suite to collect and manage information and IBM® SPSS® Statistics software to analyze the data. Survey results pointed to several areas in need of improvement – for instance, a majority of patients indicated they were insufficiently informed about where they could turn for emotional support. The hospital used its findings to make improvements and is extending its evaluation process to its nursing and outpatient departments.
5: Use predictive intelligence to drive customer interactions at every touch-point
Monitor and manage customer value
Management gurus tell us that we cannot manage what we do not measure. This is certainly true of customer relationship management. Profitable customer relationship management requires precise, timely measurement of the factors that affect customer success, and your bottom line. This effort requires a combination of historical and predictive technologies: predictive analytics, to identify customer targets for acquisition, up-selling, or cross-selling; and historical analysis, to monitor the results of marketing campaigns and sales programs.
Power CRM systems with prediction
By deploying the results of predictive analytics to every customer touch-point from your branch offices to your call center to your website, you can achieve greater effectiveness and profitability. Build predictive results into your website, and visitors will be automatically presented with the offer most likely to result in a sale. Or build predictive results into your call center, so that sales representatives know what products or offers are most likely to suit a particular customer’s needs. Every bit of data you have coming in from these systems becomes fuel for driving future customer interactions and realizing higher returns.
Want more like this?
Want more like this?
Insight delivered to your inbox
Keep up to date with our free email. Hand picked whitepapers and posts from our blog, as well as exclusive videos and webinar invitations keep our Users one step ahead.
By clicking 'SIGN UP', you agree to our Terms of Use and Privacy Policy
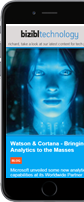
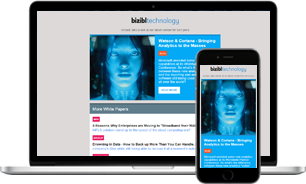
By clicking 'SIGN UP', you agree to our Terms of Use and Privacy Policy