Top 5 data sources for driving predictive marketing success
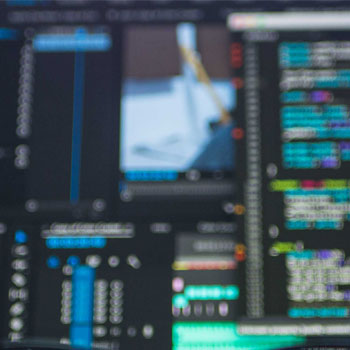
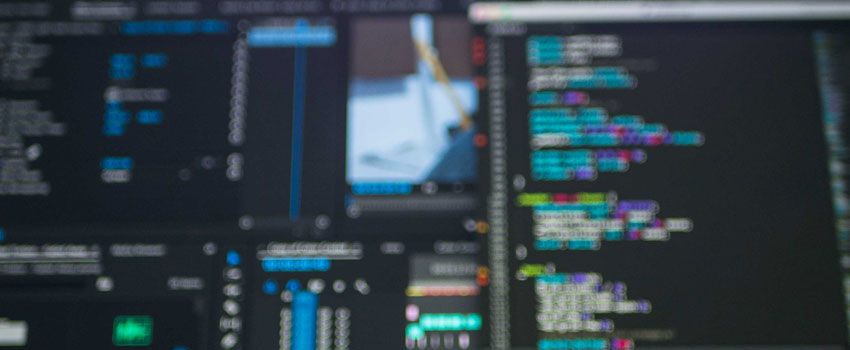
“Data is the fuel that powers AI” [Databricks]. These are seven words (AI is a word, right?) that get trotted out a lot, and that’s not a criticism - Because it is true!
When I read things like this I immediately want to know ‘what data is the most powerful?’, ‘what is the most important?’, in delivering success in Predictive Analytics. Of course, the answer depends on what the required outcome is.
RedEye is a multi-channel marketing hub, we seek to enable clients to deliver highly targeted and personalised communications across a number of channels such as email, web, social and direct mail. When we set off down this path of introducing an AI capability into our marketing automation platform, I was personally most interested in whether we could use Predictive Analytics to improve client performance across the customer lifecycle - prospects, single-buyers, multi-buyers, VIPs and lapsed. If we could use Predictive Analytics and AI to improve performance at each individual stage of the customer lifecycle, then what would be the overall impact on customer lifetime value?
We’ve had some great success - with more case studies in the pipeline. Our goal has always been to publicise any success we had, because there is a real lack of real world insight in the market around outcomes… we were keen to prove that the value was worth the investment and we believe we have - you can read about the results here with the promise that there are more soon to be released at TFM and Festival of Marketing 2018.
In this time we have learned a lot about the value of different types of data play in creating models predicting the next most likely customer action. Here is our top 5!
1. Behavioural data
I’m not messing around with a count down! Instead, straight in at the top is the most important data set! Creating a pattern of current customer behaviour is critical - on site data, email engagement, social engagement, in-store patterns, website chat data - the key is to stitch together in real time as many points of customer interaction (Behavioural Data) as possible. The ‘patterns’ created define certain likely outcomes.
2. Demographic and lifestyle data
What you know about your customer plays a part in understanding their likely behaviour, there is nothing controversial in this or that it should figure so highly in the models. The typical age, gender, location (postcode), lifestyle or life stage segment etc can all contribute significantly to predicting likely next actions.
3. Transactional data
Still a bedrock of analytics - value and frequency of previous purchases greatly influences future likely behaviour. But it goes further than that, for instance how long an individual has been a customer can figure highly in certain models.
4. Ethnographic data
Something I would previously have seen as a complete outlier, ethnographic data has figured as an important variable - surveys, offline observations, interviews, unstructured conversational data - or even the actual presence of the variable in the customer record improves likelihood to accurately predict a prospect or customers next action.
5. Data from analytics and models
Whilst I fully accept that any data on a customer is potentially important, for me this has been one of the toughest to get my head around! But we have seen how models in a single system can interact to help refine predictions. To use an example, if a customer is nearing or exceeding their predicted lifetime value, that might change the product recommendation outcome.
When I look at the last couple of years development there have been some general learnings that I have been able steal from our Analysts! The first is not to pre-judge the value of a source of data. For me it was obvious that patterns in customer behaviour, as identified through behavioural data, would be incredibly powerful. But it never occurred to me (perhaps it should have?) that length of tenure as a customer could help predict future behaviour. Or that engagement with a call centre could also influence the potential outcome, whether that engagement is positive or negative. But I was left with one over-riding perspective… allowing the system to process as much data as possible improves outcomes.
Want more like this?
Want more like this?
Insight delivered to your inbox
Keep up to date with our free email. Hand picked whitepapers and posts from our blog, as well as exclusive videos and webinar invitations keep our Users one step ahead.
By clicking 'SIGN UP', you agree to our Terms of Use and Privacy Policy
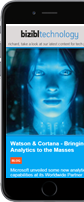
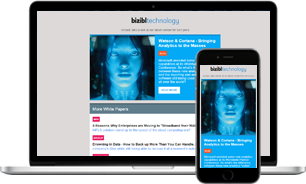
By clicking 'SIGN UP', you agree to our Terms of Use and Privacy Policy
Other content you may be interested in
Categories
Categories
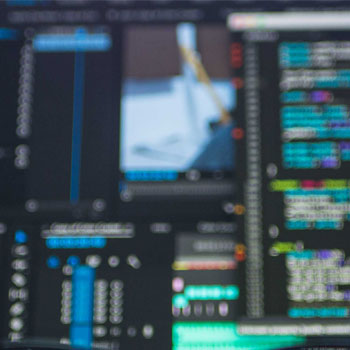
Want more like this?
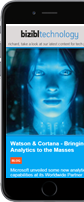
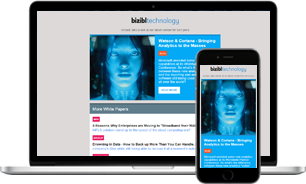
Want more like this?
Insight delivered to your inbox
Keep up to date with our free email. Hand picked whitepapers and posts from our blog, as well as exclusive videos and webinar invitations keep our Users one step ahead.
By clicking 'SIGN UP', you agree to our Terms of Use and Privacy Policy