The Fifth V of Big Data
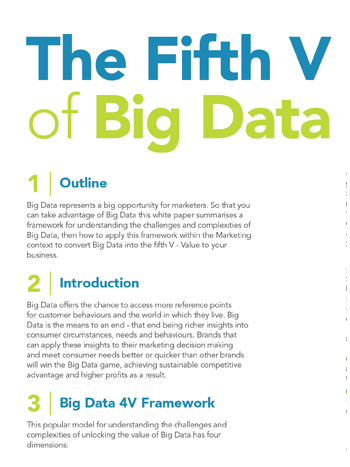
Big Data offers the chance to access more reference points for customer behaviours and the world in which they live. Big Data is the means to an end - that end being richer insights into consumer circumstances, needs and behaviours. Brands that can apply these insights to their marketing decision making and meet consumer needs better or quicker than other brands will win the Big Data game, achieving sustainable competitive advantage and higher profits as a result. This white paper summarises a framework for understanding the challenges and complexities of Big Data, then how to apply this framework within the Marketing context to convert Big Data into Value - the fifth V of Big Data.
Get the downloadBelow is an excerpt of "The Fifth V of Big Data". To get your free download, and unlimited access to the whole of bizibl.com, simply log in or join free. |
![]() |
1 | Outline
Big Data represents a big opportunity for marketers. So that you can take advantage of Big Data this white paper summarises a framework for understanding the challenges and complexities of Big Data, then how to apply this framework within the Marketing context to convert Big Data into the fifth V - Value to your business.
2 | Introduction
Big Data offers the chance to access more reference points for customer behaviours and the world in which they live. Big Data is the means to an end - that end being richer insights into consumer circumstances, needs and behaviours. Brands that can apply these insights to their marketing decision making and meet consumer needs better or quicker than other brands will win the Big Data game, achieving sustainable competitive advantage and higher profits as a result.
3 | Big Data 4V Framework
This popular model for understanding the challenges and complexities of unlocking the value of Big Data has four dimensions:
- Volume – Big Data involves enormous volumes of data. In simpler times it used to be the case that businesses created data. Now data is also generated by people (consumers) – and increasingly through things such as physical sensors, social media sites, mobile phone GPS signals and wearable devices to name a few. Every day, we create 2.5 quintillion bytes of data (Source: IBM; IBM Big Data) and that is only going to increase. (The most well known of the 4 V’s.)
- Variety – the wide range of data types and formats from structured, coded tables through to free form text and everything in between like video, images, display ad impressions, maps and articles.
- Velocity – the new sources and types of data are often the function of real time events and interactions which generate powerful insights (such as I am in the lobby of the hotel or my car has just passed a service interval) but these can only be leveraged with a rapid response to those circumstances. It’s not enough to make good decisions, you have to make them fast.
- Veracity – this is a function of the previous three Vs. The aspect of veracity is ensuring the reliability of the insights that are gained from the data. As these now have to have a context applied to them in terms of time, place and circumstance. As the volume, variety and velocity of data increases so does the challenge of ensuring quality and integrity of the data. For instance the interpretation of my willingness to book on-line and pay £200 for a hotel room would be significantly different if I’d booked same day having missed my connecting flight home from a business trip versus having booked three months in advance for a special occasion. It is not possible to create meaning without context and it’s not possible to create context without fully understanding the data that reflects the circumstances of an action, event or outcome.
The 4Vs model is a valuable framework but it is inherently technical - it’s all about Features rather than Benefits. An important counter balance to this, which helps keep a business focus to Big Data strategy and planning, is a fifth V – Value. This needs to be the key driver of a brand’s Big Data strategy and planning and the primary focus for marketers in using Big Data.
(Big) Data assets can create value for marketers in:
- Campaign optimisation – triggering and personalising customer interactions that are personalised to the customer’s specific characteristics, situation and needs
- Decision support – insights that enhance decision making across the marketing mix (e.g. pricing, budgeting, forecasting or resource allocation) that optimise the sales, marketing and service processes (for the customer and the brand) The acid test for Big Data initiatives is that they must generate pay back along either of these areas.
4 | Developing a Big Data Strategy
There is no simple answer to the question “Where is the value to be found in the available Big Data?” and Big Data practitioners warn against trying to ‘boil the ocean’ in the search for value from Big Data analytics. However applying a robust, structured and business-led approach will immediately narrow the search.
The four key steps to developing an effective Big Data strategy are:
- Data audit – identify the data you could access and consider the new customer insights that these could yield
- Customer alignment – identify the data and customer insights with greatest potential to add value to customers based on their lifecycle and purchase journeys.
- Strategic alignment - identify the data and customer insights with greatest potential to add value to the business
- Technology alignment – identify the data and customer insights you’re best placed to exploit based on your data management and analytics capabilities Using these steps take you through the process of identifying what Big Data is available, useful to the customer and to the business, and can be applied.
Step 1 | Data Audit
Marketers need to identify the different data at their disposal and understand their different characteristics in relation to the 4 Vs. The specific data available to a brand will vary from sector to sector. The Data audit step is to understand what could be available and how, when and where they could be used to create value for customers so that possible opportunities are identified.
To help some of the more common data types are shown grouped by type of data in the below illustration. The quickest wins from Big Data initiatives will typically come from those data types lower down the scale on the two axes.
Another useful planning technique is to understand the hierarchy of data available to enhance customer interactions and the decisions that these can enhance. For instance it is easier and more essential to initially understand what a customer purchased historically from your company than to understand the daily stock levels and availability that influenced the purchase, even though the latter is still very useful for marketing insight. (Identifying data hierarchy and how marketing opportunities can progressively be unlocked will be dealt with in more detail in the next whitepaper as it is a major topic in its own right.)
Exploiting quick wins via simpler, more accessible data will help marketers build proficiency and confidence in extracting and applying insights from data that enhances the customer value proposition.
The data audit results reveal:
- How data could be more fully exploited
- Where the gaps are and
- How new data could be brought into the marketing mix
As always the focus should be on fully exploiting the data already within Marketing’s reach before pursuing the returns available from bigger, less structured, more complex and disparate data (internal and external) available further from home.
If this approach is not applied then brands will miss quick win opportunities from simpler data that are easier to collate, maintain and action. This ensures early pay back with tangible, financial impact. However a subtler and even more significant benefit will be the confidence, literacy and familiarity that will result from a gradual, controlled and structured ascent of the Big Data learning curve.
For example a rail company with a loyalty scheme could look to:
- Unlock the value of transactional data to tailor and target promotions based on category purchased and channel used
- Draw loyalty scheme members into on-going dialogue in social communities relating to the brand and loyalty programme reflecting participation and advocacy in the social interactions
- Overlay external factors such as weather, customer affluence and station specific facilities with car-park capacity fluctuations and individual drive-times to the station
This measured, incremental approach will target quick wins and ensure a pragmatic approach to exploiting Big Data opportunities.
Step 2 | Customer Alignment
Customer needs and behaviours need to be the drivers for all Big Data initiatives. Consider this question: For what specific points in the customer lifecycle and purchase decision journeys will deeper insights have the most impact?
Big Data and the insights it provides must always be judged in terms of the value they create for customers. When applying the generic models above tailor them to reflect the customer experience your brand delivers. Then look through the points and explore opportunities to enhance this through greater targeting and personalisation of messages. Understanding the dynamics and timings of customer journeys in relation to the overall customer lifecycle and individual purchases represents the corner stone for developing a Big Data Strategy.
Cross-referencing these customer behaviour models with the Data Audit provides a framework for structured and rigorous exploration of specific value creation opportunities and validation that these are addressing the key areas of the customer life cycle and purchase decision journey.
For example an:
- On-line travel agent operating in a highly commoditised, competitive market with low repeat purchase rates would want Big Data insights to drive enhanced Acquisition/ Recruitment via initiatives such as optimised real-time bidding for PPC; personalised display ads and personalised landing pages for inbound web site traffic
- Automotive brand with an infrequent, high value purchase process and poor retention rates would seek to maximise repeat purchase; loyalty and in-life, service-related revenues via initiatives such as personalised, pre-emptive service plans; tailored account management from Dealer sales staff; tailored trade in and new vehicle quotes and interactive, online PR programmes via social media
The key here is to target Big Data initiatives to enhance the behaviours and experiences in the areas of the customer life cycle and purchase journey that deliver greatest value to the customer.
Step 3 | Strategic Alignment
Big Data strategy should look to ‘sweat the big stuff’ i.e. to inform and address the business questions of greatest significance to the brand. These will differ from sector to sector and brand to brand, for example a brand competing on:
- Pure play on-line will want to optimise PPC and dynamic merchandising so cookie data, tag management, promotion, pricing and product availability will be prime data opportunities
- A price-led proposition will want to optimise pricing and sales promotion so RFID tags and micro-targeting promotions based on customer location in store will be prime data opportunities
- Luxury and status will want to provide one to one interactions so in-store, personal shopper staff availability, delivery of goods for preview and monitoring of brand advocates and on-line PR coverage will be prime data opportunities
- Service levels will want to tailor service decisions re delivery, returns and post-purchase so logistics, delivery management and customer service systems data will be prime data opportunities
- Product/service personalisation will want to drive customisation so product specification/configuration and what other customers buying similar products have chosen have will be prime data opportunities
- Risk management will want to tailor credit decisions and authorisations to reflect customer needs and circumstances so the customer’s credit history and overall default rates, collections will be prime data opportunities
The data strategy must aim to support and deliver in those areas with greatest impact on the core business goals and objectives. Answering the right questions for the business, even partially, will have a bigger bearing on success than answering the wrong questions with absolute precision.
Step 4 | Technology Alignment
The data available to a business will be constrained by its technology set, systems and data architecture. These must be understood and applied as a reality check to the customer and business-led Big Data strategy that emerges from the planning process outlined in this paper.
The Data Management Platform (DMP) within the business will be the key area of technology infrastructure dictating what can and cannot be done. The new breed of DMPs are designed to operate in a Big Data environment and address:
- Real time data exchange
- Social platform data feeds
- Cookie data and tag management
- Web analytics integration
- Unstructured and text-based data management
- ERP/back office integration
- Extract, Transform and Load (ETL) utilities
- Data analytics and modelling
- Master data management and data hierarchies
- Mobile integration
Reconciling technology capabilities with business requirements is an increasingly important part of the Big Data planning process.
5 | Conclusions
Big Data is broad and amorphous topic and is now a generally understood concept which marketers acknowledge as holding huge potential. The challenge is to translate aspiration into operational reality and make the new data sets work hard for consumers and brand.
There is no magic wand here. But equally with the right support and commitment marketers are well placed to use the simple frameworks and methodologies outlined in this white paper to lead a Big Data planning process which is grounded in the realities of their customers, products and brands to:
- Audit current data sets
- Relate these to current CRM programmes and campaigns
- Identify gaps in the use of existing data
- Spot new, ‘killer’ insight opportunities from new data sets
- Prioritise Big Data initiatives based on:
- consumer needs
- business strategy
- Reconcile Big Data strategy to technology capability
The aim is to empower marketers to lead the Big Data strategy and planning process. This, like CRM and customer experience strategy development has to be a multi-disciplinary, enterprisewide undertaking but marketers must lead the way. Not because of any political or organisational hierarchy agenda but rather to ensure that the customer, and serving them better, lies at the heart of Big Data strategy and the multitude of initiatives it will be made up of. Zeta Interactive is a leading Customer Lifecycle Marketing company that uses Big Data and proprietary technology to acquire, engage and retain customers. For more information on gaining competitive advantage from Big Data please contact Zeta Interactive on the below number.
Want more like this?
Want more like this?
Insight delivered to your inbox
Keep up to date with our free email. Hand picked whitepapers and posts from our blog, as well as exclusive videos and webinar invitations keep our Users one step ahead.
By clicking 'SIGN UP', you agree to our Terms of Use and Privacy Policy
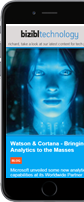
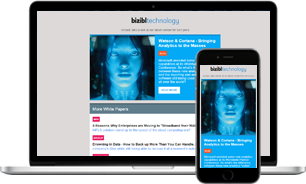
By clicking 'SIGN UP', you agree to our Terms of Use and Privacy Policy