Data Quality: Reality and Rhetoric
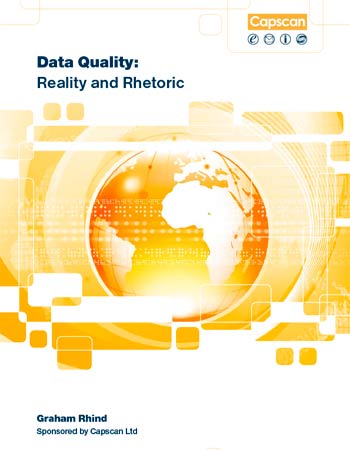
Incorrect, incomplete or ‘dirty’ address and contact data incurs unnecessary costs on the business in terms of wasted time and money. It not only impacts on profit margins, but can also damage brand image and increase a business' exposure to identity theft and fraud. This whitepaper surveys the opinions of international businesses and examines how effective data quality management (DQM) can: Improve operational efficiency and international marketing, prevent data decay and improve data accuracy & customer service and enhance data analysis and help you achieve a single customer view
Get the downloadBelow is an excerpt of "Data Quality: Reality and Rhetoric". To get your free download, and unlimited access to the whole of bizibl.com, simply log in or join free. |
![]() |
Introduction
In 2008, Capscan commissioned a survey* to measure the perceptions that companies have about data quality and the actions that they are taking to achieve improvement. It found that, whilst people are increasingly aware of data quality, a gulf remains between perception and action. Few companies had the policies, tools or processes in place to tackle poor data quality effectively, and companies hugely overestimated the quality of their in-house data.
Has there been improvement since that survey? Has the changing economic and regulatory environment given impulse to changes within organisations’ data quality regimes? Is action catching up with intention? To answer these, and other, questions, Capscan commissioned MCC International Ltd to repeat its data quality management research from 2008 amongst the readers of CBR Online. 220 completed questionnaires were received. The readers are skewed towards higher management, particularly in information technology, within large organisations and mostly within the United Kingdom.
Though the profile of the respondents is slightly different to those of 2008, in many cases similar results were produced. Some of the minor differences in results may be put down to these profile differences. The results showed a great deal of similarity with the 2008 results, suggesting that change and improvement is not happening as quickly as necessary
Key findings
Responsibility
Defining data ownership, stewardship and responsibility are perennial problems within most companies, with departments claiming data for themselves and few companies having an enterprise-wide view of the issues involved. Often, companies see data quality as a technical issue with technical solutions and they place responsibility squarely with their IT departments. This survey supports this continued trend. 30.4% of respondents name members of the IT department as responsible for data quality (as against 26.6% in 2008); 10.5% name upper management (11.2% in 2008), 17.7% name leaders within the marketing department (22.3%) and 5.9% name leaders within sales departments (5.9%).
Many data quality issues will not be identified or resolved, however, whilst responsibility is placed within a single department or with a single individual. Almost every person within an organisation, and certainly all of those who contribute to, and consume from, data resources, must understand the importance of maintaining data quality to the future of their company, and their part in that process. Business structures that name a single person, role or department as being responsible for data quality allows other staff to point at them when problems arise – an “it’s their problem” attitude – which will always lead to a lower level of data quality than when all staff are part of the data quality process. In 2008 only one respondent recognised this reality. Encouragingly, the 2010 survey showed 4 respondents recognising that everybody has responsibility for data quality, it being a collective responsibility.
The preponderance of higher managerial positions named as responsible does, however suggest that data quality is being viewed as something of sufficient importance to merit the attention of senior management.
Words and actions
The contrast noted in 2008 between words and actions in many organisations remains an issue in 2010. There seems to have been a slight reduction in the number of respondents with an enterprisewide data quality management policy in place – 38.8% (against 41% in 2008), with 46.6% stating that no strategy was in place (40.4% in 2008). The discrepancy remains that 87.3% of respondents named a staff position as responsible for data quality and 88.6% stated that data quality within the organisation was fairly or very important, suggesting that whilst data quality is viewed as important by many respondents, the integration of data quality practices into the daily life of those companies is not common and there is a great deal of room for improvement at a strategic level.
Want more like this?
Want more like this?
Insight delivered to your inbox
Keep up to date with our free email. Hand picked whitepapers and posts from our blog, as well as exclusive videos and webinar invitations keep our Users one step ahead.
By clicking 'SIGN UP', you agree to our Terms of Use and Privacy Policy
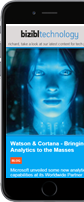
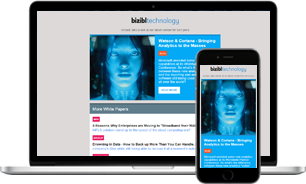
By clicking 'SIGN UP', you agree to our Terms of Use and Privacy Policy
Though it may be the case that a person has that responsibility named within their job description, without a data quality management policy in place, little effective data quality process improvement is likely to take place. Furthermore, the use of software and processes to actually improve and protect data quality is relatively low.
Data quality processes
Some processes, such as de-duplication, especially during times of economic stress, are seen as a quick win for improving data quality and decreasing costs, and this is borne out by the survey. An increased 36.2% of companies (32.4% in 2008) use de-duplication software. It remains a concern, however, that this leaves 64% of companies with duplicate-ridden database, a massive burden and cost-base for the organisations and their customers alike.
Address validation for United Kingdom data is also seeing a healthy increase, being used by 41.6% of respondents as against only 28.7% of those who responded in 2008. International address validation, however, fares less well, being used by 12.8% of companies (17% in 2008). This may be explained by the decreased number of respondents from outside the United Kingdom in 2010, and suggests that companies within the UK either only have customers from their domestic market, or are largely not giving the same attention to the quality of data from their international customers as they are to their national customers.
Apart from international address management software, penetration of other quality products showed an improvement across the board from 2008.
Almost 85% of the respondents collect name and address information, but only about 40% of those state that they validate this information using software, significantly down from 2008 and illustrating a major source of poor data quality and a large potential market remaining for data improving software and procedures. It is difficult to square these results with the apparent increased penetration of data quality products into the organisations which responded to the survey. There is also a clear and significant gulf between the appreciation of the importance of data quality and steps having been taken to put into practice processes to ensure that high data quality is achieved. This may in some part be due to the continuing necessity for those working with the data to persuade upper management of the need to take steps to assure data quality.
The discrepancy between collected versus validated data is shown in the graph above, and it illustrates that although businesses purport to recognise the importance of data quality, they have yet to implement the systems and processes to ensure its reality. The gap between recognition and action remains disappointingly wide.
The politics of data
The continuing necessity to persuade management of the requirement to improve data quality, and to prove return on investment on something that is intrinsically obviously advantageous to any company, is shown in the survey’s results. 25% (27.7% in 2008) of respondents stated that “establishing a link between data and ROI”, 30.5% (31.4% in 2008) “Getting senior management buy-in for a data strategy” and a massive 36.8% (39.4% in 2008) “Getting organisations to understand the impact of poor data” were seen as some of the biggest data challenges today by the respondents. However, encouragingly, focus is increasingly being put onto the data itself. 68.6% of respondents are being challenged to ensure that data is up-to-date and accurate, and 52.3% to ensure that data is secure and well-managed. Accurate data is the basis for any successful use of the data for information purposes, and any work put into these aspects of data management will never be wasted.
Optimism
A major barrier to better data quality is an eternal optimism that many companies and their staff have about their data and its quality. Companies will be unable to overcome inertia in improving data quality if they cannot recognise that their data has quality issues, nor gain the advantages that they will accrue from improving that quality
Without recognising the problems and issues causing poor data quality, organisations will not tackle these issues and other data quality issues which affect the health of that organisation will remain extant. Staff members are eternally optimistic about the quality of the data being held and managed within their organisations.
Want more like this?
Want more like this?
Insight delivered to your inbox
Keep up to date with our free email. Hand picked whitepapers and posts from our blog, as well as exclusive videos and webinar invitations keep our Users one step ahead.
By clicking 'SIGN UP', you agree to our Terms of Use and Privacy Policy
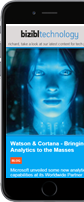
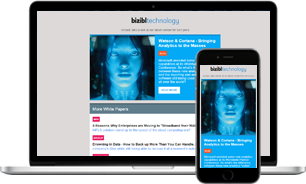
By clicking 'SIGN UP', you agree to our Terms of Use and Privacy Policy
This has been well illustrated in this study. 57.7% of respondents (up from 52% in 2008) viewed their organisation’s data as being of excellent/good quality, and a further 34.5% (40% in 2008) considered their data to be “alright – but could be better”, leaving only 7.7% (8% in 2008) to admit that their data was poor. Given the relatively low number of respondents utilising validation and cleansing software such as de-duplication and address validation, it is clear that the quality of data is being grossly overestimated. This may partially be due to the data being hidden behind complex programs and interfaces, reducing the ability of the users to actually view the data outside reports where data is coalesced into a set of figures; and also due to most data professionals not being able to recognise problems in their data even when they are able to browse through it. Data needs to be collected in as clean a way as possible, with validation at source, and it will often start to decay even before it enters the database. Many of the 92.2% of respondents assuming that their data is “alright” or better are usually oblivious to the reality of data quality issues.
Perceptions
As in 2008, respondents are better able to see the positive effects of good data quality than the negative effects of poor data quality, with each respondent naming on average one more positive point than negative point. Respondents see negative aspects largely in terms of the direct internal effect on their business and on themselves. Factors such as operational inefficiency, loss of revenue opportunities, increased costs and the extra workload to check and correct data score highly. The effect of poor data quality on the real owners of that data, their customers, is less obvious, with fewer than half of respondents identifying increased customer dissatisfaction as a negative impact, and only a quarter identifying loss of trust in the company.
Interestingly, “Making data compliant with regulations and standards” received a lower score in 2010 (37.3%) than in 2008 (47.9%), despite the increasing importance of regulation within data quality and the financial stresses that many organisations currently find themselves in. Many companies are behind in the curve in making themselves compliant to the increasing store of data and privacy regulations.
As was the case in 2008, a general optimism about data quality affects the answers to questions about data quality’s affect on the business. One would expect the same number of respondents recognising that good data quality will increase operational efficiency to recognise that poor data quality will decrease operational efficiency. In fact, more positive effects were noted that negative effects, with the difference reaching as much as 15%.
Who’s to blame?
In 2008 it was clear that organisations were keen to shift any perceived blame about data quality to the front-end, to their customers and to their own staff.
It is therefore encouraging that this perception is less marked in the 2010 survey. 24.5% of respondents (as against 33.3% in 2008) blamed “inadequate data entry by customers” as one of the main sources of their data quality problems. Far more, 58.2% (but down from the 65.4% in 2008) blamed inadequate data entry by employees. In both cases, the organisation has the power to improve results through technological implementation (through, for example, validation software) and procedural business process improvement and training. A great deal of control can be placed on data entry systems through the use of dynamic forms, validation, dialogue with the customer and a better analysis of data being gathered. These are problems which companies could begin to address today and which could have major positive effects on data quality.
Taking over as the greatest source of data quality problems has been “data decay over time”. Data needs to be collected correctly, and some data, such as data of birth, does not decay. Other data, such as personal names and addresses, decays because the real-life entity to which the data refers changes in some way – a person changes their name upon marriage, for example; people move, new houses are built, others are demolished. In all of these cases work is required to ensure that data is kept valid – through validation software and also through effective dialogue with the customers to allow their data to be managed and maintained to the highest possible level.
Spending is flat
Overall spending on IT initiatives has been largely flat, with just over a third (35.5%) of respondents reporting no change in their spending, about a fifth (20%) reporting a rise and a similar number (22.7%) reporting a fall in spending. Spending on data quality IT initiatives (Customer Relationship Management, Master Data Management and Data Quality Management) are generally not outstripping general IT spending, and a similar pattern is expected for spending in 2011.
Although data quality initiatives are not (and should not be) always IT-based, as a great deal of data quality improvement can be achieved through, for example, better business processes; given the major gap that this survey has exposed between actual and perceived data quality within organisations, the lack of data quality initiatives in place and a general failure to address both data quality issues and regulatory requirements related to data quality, spending on effective data quality improvement clearly must increase. Cutting back on data quality is not an option.
Conclusion
A comparison of the 2008 and 2010 survey results suggest that there have been no great strides forward in the management of data quality within many companies. The 2010 findings reveal a mixed picture. Whilst many organisations recognise the importance of data quality management to a business, its penetration amongst all organisations as a strategic agenda is still not high. Furthermore, too few have the necessary processes, tools or strategic will to do something about it. Contact information collected by organisations is, in many cases, still not checked or validated, and data is not measured or financially valued enough at a strategic level. When we compare this year’s results to those of 2008, we find that generally the environment for improving data quality has not improved. The financial crisis may have affected spending on IT initiatives, leading to more conservative investment patterns. Organisations do not truly understand the benefits of data quality management (DQM) and its ability to help organisations achieve a single customer view and better strategic marketing. For most organisations DQM is about operational improvement and not something of true strategic relevance. The gap between the organisations stating that they had a data quality management strategy and realising it is still fairly large. Reality still lags behind the rhetoric.
Want more like this?
Want more like this?
Insight delivered to your inbox
Keep up to date with our free email. Hand picked whitepapers and posts from our blog, as well as exclusive videos and webinar invitations keep our Users one step ahead.
By clicking 'SIGN UP', you agree to our Terms of Use and Privacy Policy
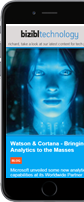
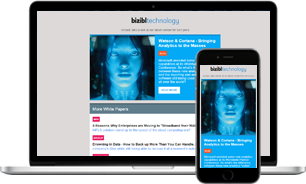
By clicking 'SIGN UP', you agree to our Terms of Use and Privacy Policy