Use This Lever Effect to Get Powerful Insights from 1st Party Data
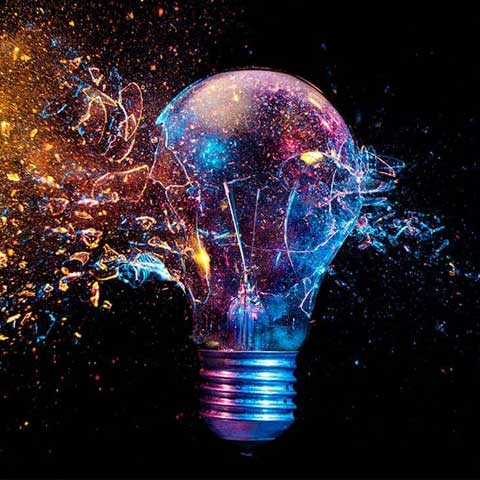
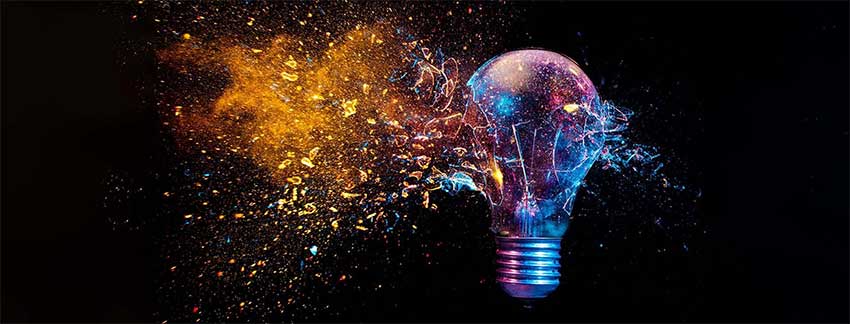
With the well-publicised demise of the use of third-party cookies for marketing purposes, companies are having to re-think their data and marketing strategies. Enter zero- and first-party data ‘centre stage’. Here's what you need to know about maximising the value of first-party data.
Zero-party data is data that customers have willingly shared with companies, usually in exchange for something (e.g. contact details in exchange for a whitepaper, personal preferences or survey responses). First-party data is data about a company's customers that is collected and owned by that company. It is typically created as a result of transactions or interactions in the course of daily business (e.g. CRM or webshop data) and can be used to create insights to drive your marketing decisions.
Limited value
The problem that marketers face is that as it is collected and stored in operational systems, zero- and first-party data only has limited value in terms of insight creation. Say you have 20-50 data elements coming from your upstream operational systems (CRM, webshop, email, etc.) including data such as contact details, channel permissions and transactional data such as date of purchase, purchase amount and product purchases. Smart marketers can create dozens and in some cases hundreds of new, so-called ‘derived’ marketing variables from this source data.
An Apteco partner recently shared with me how they generate 800 variables from 35 input data fields. This is how powerful insights are generated. This capability allows companies to lay the foundation for a sustainable competitive advantage via enhanced customer experiences. The more first-party data you have access to, the greater the potential for insight generation.
To create these new derived variables, marketers need three things:
- Direct access to their marketing data. Surprisingly, many companies stumble at this first hurdle.
- A comprehensive set of data manipulation functions (comparable to Excel functions) that work on a record-by-record basis to return a new value, derived from variables and functions that act on them. There are literally hundreds of functions that add value to marketing data.
- An easy way of using these new values in analyses or even turning them into permanent variables that marketers can work with on a day-to-day basis for targeting, analyses, and campaign personalisation. The variables are automatically updated as fresh data comes into your marketing automation system, allowing, for example, communications to be triggered by changes in values.
These data manipulation functions can range from very simple to highly complex.
Simple to complex variations (without relying on IT)
A simple function could be used, for example, to transform a date-of-birth into a categorised data range variable (e.g. ‘30-40 year olds’). A more complex expression could be used, for example, to identify a customer’s favourite product category across all purchases. From a marketing perspective, the value of this ‘new’ derived data can be enormous. For example, calculating the number of days since the last purchase and subtracting the average number of days between purchases for each customer can provide a useful indicator of possible churn. The use cases are many and varied.
Some of you reading this may be rolling your eyes and thinking: “But that’s the job of our IT department”. WRONG. Marketers are the ones who are closest to the marketing data and they are the ones with the ideas for new variables. They should have the flexibility to create hypotheses and test them out – quickly. If you leave it to your IT department, you will end up with a list of static fields that don’t change and are very expensive to create and maintain. If the creation of a new variable becomes an IT ‘project’, it is hard to operate as an agile marketer in today’s competitive marketplace, where new data sources and data fields are constantly becoming available.
A marketer may, for example, suspect that there is a correlation between the distance from the customers’ home addresses to your local department store and lifetime value. In order to prove or disprove this hunch, you want to be able to quickly calculate customers’ distance from their nearest store, maybe banding them into meaningful categories (e.g. 0-15 miles, 15-30 miles and 30-45 miles). You may want to be able to show these correlations in an analytic cube or visualisation.
Here are some common use cases for calculating new data from your first-party data.
Date and time-based derivations
These may include, for example:
- Anniversary calculations (e.g. six months or 1 year from first purchase)
- From a sequence of historical purchases, calculate what the Next Best Date (or time window) is for contacting a customer
- Calculating the average number of days between receiving a marketing communication and making a purchase, or between a call to the service centre and cancelling a contract
Geo-based derivations
These may include, for example:
- What is the distance between each customer and their local store? Is this the same as the store they actually purchase from?
- Distance to nearest airport or train station. How does this impact their choice of holiday destination?
Pattern analysis derivations
These may include, for example:
- What is the customer’s ‘personal best’ (value, size, performance) and whether the customer is growing or declining over time.
- What is the longest sequence of identical products bought?
- What is the sequence of events prior to an outcome e.g. mailing -> online ->store -> online -> purchase.
Aggregation-based derivations
Aggregation of transaction data from one marketing ‘level’ to another over-arching level (e.g. individual purchases over time to a single customer lifetime value).
These may include, for example:
- In the energy sector: aggregating meter readings to individual gas or electricity contracts and then aggregating contract values to a total customer value.
- Email clicks aggregated to a customer total, allowing a digital engagement score per customer to be calculated.
- Recency, Frequency and Monetary (RFM) value aggregations.
Based on each company’s business model, the marketer will soon identify dozens if not hundreds of new variables, which can then feed into the analysis process to create meaningful insights. Only then will first-party data really start to drive your decision making and your marketing success.
The Apteco approach
Rather than rely on a fixed data model and fixed pre-defined insights, Apteco takes a different approach and supports a completely flexible data model, allowing easy addition of new data sources and variables. With over 200 out-of-the-box functions (and new ones being added all the time), the marketer can quickly start carrying out easy and fast marketing calculations from the source data and convert these to new permanent variables that can provide powerful new insights to drive your marketing communications.
If you’d like to find out more, ask for a demo of Apteco FastStats to see how to leverage your first-party data, or try a free-trial of Apteco Orbit, where all your insights can be visualised in dashboards to drive your marketing campaigns. At Apteco, we call this “Insight into Action”.
Want more like this?
Want more like this?
Insight delivered to your inbox
Keep up to date with our free email. Hand picked whitepapers and posts from our blog, as well as exclusive videos and webinar invitations keep our Users one step ahead.
By clicking 'SIGN UP', you agree to our Terms of Use and Privacy Policy
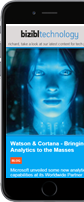
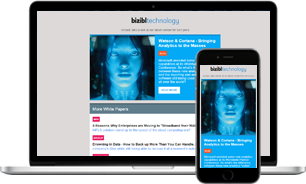
By clicking 'SIGN UP', you agree to our Terms of Use and Privacy Policy
Other content you may be interested in
Categories
Categories
Categories
Categories
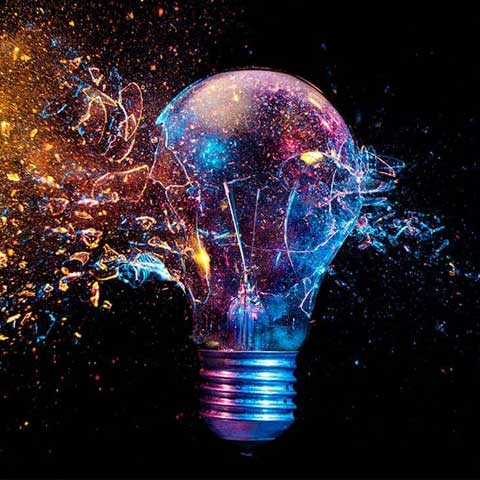
Want more like this?
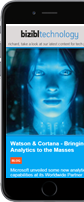
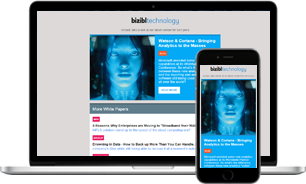
Want more like this?
Insight delivered to your inbox
Keep up to date with our free email. Hand picked whitepapers and posts from our blog, as well as exclusive videos and webinar invitations keep our Users one step ahead.
By clicking 'SIGN UP', you agree to our Terms of Use and Privacy Policy