Out of the Dark Ages: The Rise of Social Media Sentiment Analysis
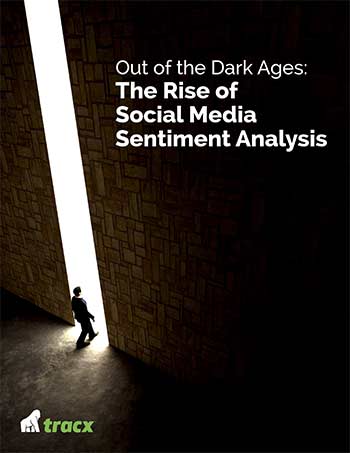
Over the past 5 years, sentiment analysis has become an increasingly integral part of social media management for enterprises of all sizes. No matter what industry or vertical an organisation may be in, it is imperative to gain a secure hold on how people and consumers are receiving company messaging and product information. This whitepaper provides a debriefing of the history of sentiment analysis in social media, as well as a preview of how sentiment tracking engines are evolving to fulfill the increasing demands of the industry.
Get the downloadBelow is an excerpt of "Out of the Dark Ages: The Rise of Social Media Sentiment Analysis". To get your free download, and unlimited access to the whole of bizibl.com, simply log in or join free. |
![]() |
In The Beginning…
During the past two years, the social media industry has experienced incredible growth in the scoring and functionalization of sentiment analysis. Sentiment can be defined as a view or attitude towards a situation or event, and refers to people’s feelings and opinions on a given subject. Measuring and quantifying sentiment surrounding an industry or brand is essential for an organization to gain a secure hold on how people are truly receiving a brand’s messaging, products, or overall voice.
Beyond The Obvious
By looking at obvious numbers such as the amount of company page likes, number of followers, and volume of interactions received on owned media content, brand managers would compare their social impact with their competitors’ numbers. But the industry would soon learn that high-level statistics such as follower counts are often irrelevant and misrepresentative, as someone can follow a company on social media without showing any further interest in what that company is saying or doing.
Beyond the inaccuracy of only looking at non-descript statistics, during the corporate social media Dark Ages the approach to measuring sentiment was one plagued by the use of solicitation. Brands would openly and directly ask consumers and people with interest to an industry about their sentiment regarding brands and campaigns via surveys and focus groups. While this was thought to be useful at the time, and insights into general audience reception were yielded, with this method comes the unavoidable obstacle of overcoming biases.
The Technology Bottleneck
Eventually, managers within the enterprise came around to recognize the importance of measuring and recording the sentiment of the individualized, consumer-produced posts surrounding their brands. This process was excruciatingly manual, as a trained representative would have to physically dig through each post and tag it as having positive, negative, or neutral implications. There were no text-analysis algorithms available that could accurately score the sentiment of posts, and therefore no easily obtainable and summarizing reports to pass up to the C-level.
It wasn’t until later that the process of sentiment scoring, recording, and reporting became more automated and streamlined, and social media sentiment analysis emerged from The Dark Ages into The Renaissance, where the technology began to catch up to the market’s needs…
The Rennaissance
During The Renaissance, sentiment analysis in social media was manual, slow, inaccurate, and limited heavily by the technology readily available in the marketplace. But things were changing rapidly…
Once the concept of sentiment analysis had been legitimized and adopted throughout the social media industry, significant strides were made to automate the scoring, recording, and reporting processes. The biggest enhancement of sentiment analysis rested on the shoulders of machinelearning algorithms.
The Rise of Machine-Learning
By 2013-2014, algorithms being utilized by major SMMS platforms yielded about 70% accuracy when it came to identifying posts as positive, negative, or neutral. If an organization wanted to increase the accuracy beyond that, a skilled representative would train the system by sorting through a significant number of social posts, and manually scoring them until the algorithm had enough data to refine its definition of positive, negative, or neutral sentiment.
The new wave of machine-learning was significant for the evolution of sentiment analysis, as algorithm-based sentiment scoring only grew more accurate depending on the number of manually adjusted post scores. Analysis at the post level meant that each piece of content received an overall score. While a post may have been comprised of both positive and negative expressions, only one definitive social media sentiment score would be applied, thus limiting an organization’s understanding of consumers’ true perception of their brand.
Limitations: An Example
In the following example Tweet, we address the limitations surfaced by early machine learning.
At this time, the limits of generalized sentiment analysis were consistently present, as there were only three levels of scoring – positive, negative, and neutral. As shown in the example above, social posts can express mixed tones that would be misinterpreted by traditional sentiment tracking engines. A traditional system would see the words “wonderful,” “thanks,” “better,” and “love,” and could ultimately score this post as positive, when in reality it is actually expressing a very mixed experience (both positive and negative). The majority of current social sentiment tracking systems can’t process this type of content. Further, if the post had been measured manually, the user would have to either assign an inaccurate overall score, or simply ignore the post.
Want more like this?
Want more like this?
Insight delivered to your inbox
Keep up to date with our free email. Hand picked whitepapers and posts from our blog, as well as exclusive videos and webinar invitations keep our Users one step ahead.
By clicking 'SIGN UP', you agree to our Terms of Use and Privacy Policy
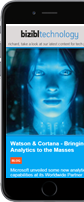
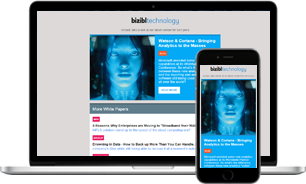
By clicking 'SIGN UP', you agree to our Terms of Use and Privacy Policy
Brands Need More Control
I n addition to the language limitations, brands needed a way to categorize sentiment across different categories that were important and unique to their organizations. The majority of platforms on the market only provided basic machine learning sentiment coupled with limited filtering functionality. Brands demanded greater granularity and segmentation of sentiment data. They needed a structured framework to address sentiment in key areas that each brand needed to measure. For instance, a CPG brand would benefit from looking at packaging, taste, ingredients and other categories, while an automotive company would have far different categories of interest such as comfort, handling, service, and various others. The generalized sentiment score did not tell an accurate story, at least not until that score could be broken down further.
In addition, many of these tools were standalone offerings that required jumping between different platforms, pulling from disparate data sets. While this was a partial solution, sentiment without context was still inaccurate.
But these hurdles would soon be obsolete…
The Enlightenment
The desire to measure social sentiment at a more granular level has risen rapidly within the industry. How do you gauge the sentiment of a long-form post or even a Tweet conveying mixed signals? How can you group terms and phrases together to gain a greater understanding of sentiment within unique and flexibly defined categories relevant to your industry and company?
A better, more accurate scoring mechanism was needed. That mechanism is Sentity.
The Introduction of Sentity
The process of scoring, recording, and reporting sentiment on social media has been changed dramatically with the introduction of Tracx’s Sentity engine. Sentiment classification is no longer based on language cues across the entire post, as users can now define specific keywords and phrases for sentiment tracking called “entities.” With Sentity, greater accuracy and deeper analysis are gained by looking at smaller pieces of language surrounding each mention of these user-defined entities. In addition, entities The Introduction of Sentity Entities are defined as a term representing a single person, place, or thing, about which data can be stored. The Enlightenment can be grouped into larger categories for greater insights into key focus areas for brands.
Entities are terms representing a single person, place, or thing, about which data can be stored. Entities maintain distinct and separate existence and objective, can be classified, and have stated relationships to other entities. This shift in focus from entire pieces of content to individual entities within each post allows users to analyze the sentiment of multiple terms and phrases within a single piece of content to uncover truly actionable, focused insights.
Example: How Sentity Creates Greater Accuracy
For instance, in the example previously examined (see above), a traditional system would see the words “wonderful,” “thanks,” “better,” and “love,” and could ultimately score this post as positive. This method ignores the evident sarcasm and other influential pieces of the post such as the “service getting better” or the “love” for the legroom.
The Tweet expressed a very mixed sentiment that simple sentiment scoring engines would measure incorrectly, and the weight of each measure would become subjective depending on the focus/needs of the brand. Accordingly, the final sentiment score would be skewed. With Tracx’s Sentity engine, granular measurement of sentiment at the entity level becomes possible, producing a highly accurate sentiment score of the post and any key entities being measured.
More importantly, by layering in the use of definable entities, and by tagging the “delayed flight” portion of the post as having a negative sentiment within a “flight status” entity, an organization can then start to gain deeper insight into more granular categories. The Sentity engine provides easy measurement into key areas of a brand, not just an overall score. The above-cited example could have contained more complex messaging, and actually could have been tagged across multiple entities based on user-defined criteria, yielding highly accurate sentiment scores directly aligned with an organization’s KPIs.
By compiling a complex sentiment score through identifying specific entities within the entire post, generalized and inaccurate classifications of expressive tone across a whole post become a thing of the past… Good riddance to vague and inaccurate methods!
Sentiment analysis prior to Sentity measured content on an extremely broad level via an overall score of the content within a post. Scores did not accurately reflect the true social media sentiment surrounding a brand or term. Sentity surpasses this limitation, as different words and phrases within a post, or “entities,” are measured individually for sentiment. Then, these “entities” are further categorized into user-defined focus categories, creating more accurate and reliable sentiment measurement.
Sentity allows sentiment tags, and the overall breakdown of data, to be more fluid and customizable. Sentiment scores can now be immediately pivoted and sorted based on the entities defined by the user. The sentiment criteria is not locked, so it shifts dramatically depending on what data the users choose to focus on. For instance, CPG brands and automotive brands have distinctly different entities / categories that they wish to focus on and measure more acutely.
A Unified Approach to Social Media
Sentity gives users across multiple departments the power to adjust the sentiment association of terms and phrases, substantially increasing accuracy to reveal a level of insight previously unavailable to the market. As part of the Tracx platform, these insights come with a unified, holistic view of the entire social media ecosystem, including demographics, analytics, listening, customer care, crisis management, customizable reporting, and real-time engagement. Industry leading analytics and engagement now includes the industry-leading sentiment analysis engine, Sentity
[Download PDF to see Checklist]
Want more like this?
Want more like this?
Insight delivered to your inbox
Keep up to date with our free email. Hand picked whitepapers and posts from our blog, as well as exclusive videos and webinar invitations keep our Users one step ahead.
By clicking 'SIGN UP', you agree to our Terms of Use and Privacy Policy
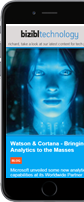
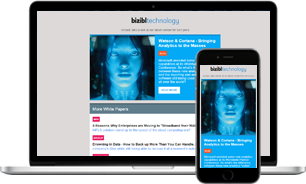
By clicking 'SIGN UP', you agree to our Terms of Use and Privacy Policy