How to Deliver a Smarter Retail Experience
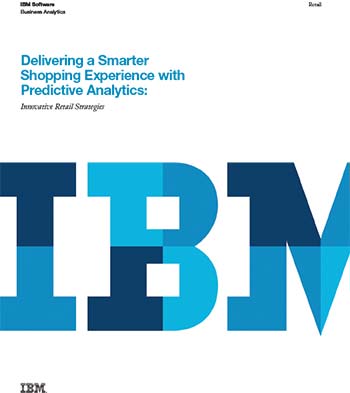
Today's consumer has more power than ever before. The rise of Internet enabled mobile devices has allowed shoppers to instantly access a wealth of information about retailers and their products. Retailers now need to deliver a smarter shopping experience in order to differentiate themselves. This whitepaper explores the practical applications of predictive analytics within retail and how it can be used to deliver a smarter shopping experience.
Get the downloadBelow is an excerpt of "How to Deliver a Smarter Retail Experience". To get your free download, and unlimited access to the whole of bizibl.com, simply log in or join free. |
![]() |
Data Drives the Experience
Any retailer hoping to keep pace with today’s consumer requires foresight and insight. They must consolidate data and optimize operations for quick decision-making around marketing, promotions and merchandising — often in real-time, to successfully obtain and retain customers.
And as retailers learn more about their customers, they can build a wider, more complete picture of the desired experience. Imagine customers browsing a retail site in search of items they might be interested in or need. This leaves a lot to chance. But by leveraging data derived from behavior and trends, retailers drive the experience, navigating customers to products based on predictive models. This allows for a better shopping experience but also one that can be further extended to include a personalized and real-time delivery.
Personalized Mobile Marketing
Nothing embodies consumer empowerment more than the use of mobile devices. Forward-thinking retailers are devising mobile strategies to address each stage of the consumer cycle — awareness, engagement, consideration, conversion and loyalty. Mobile apps create unique opportunities to engage with consumers, whether in a store, in transit or online. For example, messages can be tailored to a customer’s profile based on predictive analytics models, plus information captured on his or her shopping habits. Targeted products can then be offered through personalized messages specific to that customer — creating a win-win for both customer and retailer.
How does this look in practice? Let’s say someone opts-in to receive marketing messages from a retailer who has an outlet in the local mall. GPS-integrated tracking detects that the customer is in close proximity to the store and sends the customer a text message alerting them to a special one-day offer. The offer is driven by what the retailer already knows about this particular customer, based on profile, loyalty card data, stated attitudes and predictive analytics modeling. With the customer’s interest piqued they head into the store and purchase using the coupon code in the text message. Rather than assuming just that single purchase, the text message also alerts the consumers to another offer — an item that customers like them tend to purchase in concert with the first item (like a shirt and a tie).
In short, consumers are getting smarter in their use of technology and this is likely to increase their expectation for retailers to keep up
What Does the Research Reveal?
In a recent IBM study involving more than 1500 CEOs in 25 countries, 93 percent of retail CEOs surveyed considered it a top priority to “better understand, predict and give customers what they really want.”
The challenge lies in enabling a seamless shopping experience, one that aligns the right products with the right placement, ensures consistent cross-channel promotions and delivers timely and relevant communications. There is no shortage of data; it pours in from multiple systems, channels and regions. Retailers also generate huge amounts of transactional information containing a wealth of detail on purchase patterns. If used properly, this data can help retailers move towards a new era — one where keeping pace with consumers’ needs and having insight into the patterns pays big dividends.
By extracting meaningful analytics from the data, retailers can ensure they are maximizing their marketing efforts by targeting the right consumer with the right offer at the time — making each interaction unique and profitable. Having insight into patterns, products and promotions enables the retailer to match shopper preferences and behavior as well as provide maximum return on their marketing spend. To facilitate ROI, retailers need to understand:
- Product sales patterns and buying preferences to increase sales and margins
- Which products — or product combinations — are most likely to drive customers to purchase
- How to increase marketing effectiveness and retain the most loyal customers to maximize profitability
- How to personalize marketing and customer experience to individual shoppers
Predictive Analytics Takes Retail to the Next Level
Retailers are already using some degree of analytics to determine price elasticity, spot trends, address seasonality and report on the progress of different channels and regions. Predictive analytics adds the leverage to transform retail organizations. The maturity model described later in this whitepaper gives a practical way to gauge where a retail organization is today and where that organization has the potential to go in the future. Simply stated, applying different analytic techniques enables retailers to differentiate a shopping experience against that of competitors.
For those of us fortunate enough to have had the experience of a personal shopper, we typically get more items than we had initially planned. The experience is enjoyable — no waiting in lines or searching for items that might or might not work. Best of all, it’s done by a professional who knows our preferences. We associate personal shopping with the fashion industry, but with predictive analytics personal shopping experiences becomes a reality for most consumers.
When a retailer looks at the products people buy together, valuable insights can be learned. And analyzing segments or clusters of people delivers even bigger rewards. Retailers who have close relationships with customers, for example, through loyalty programs or online shopping registration, can refine their approach by including a wider set of data to the models they are building. This allows for offers based on specific customer profiles. This means customer data can be highly targeted and focused on an individual so offers are informed by lifestyle preferences or a key event such as a pregnancy or new job.
In addition to transactional data, when modeling customer behavior, retailers can use other types of data to amplify the personalization effort, such as:
- Descriptive data — combining self declared data with any bought in geo demographics based on Zip or postal codes
- Interaction data — information that describes how customers interact with retailer’s online portals such as ecommerce and loyalty club website, or customer service centers
- Attitudinal data — information that describes why consumers do what they do, attitudinal survey data or information collected via social media such as blogs or forums
What’s in Your Basket?
Retail operations generate a vast amount of Point of Sales (POS) transactional data, recording exact details of every purchase through a store or website. Predictive analytics lets retailers take all this data and determine underlying patterns (associations) and calculate primary, secondary and tertiary recommendations for that individual customer or customer segment. For instance, in applying market basket analysis, algorithms can reveal associations between products that are typically bought together. Key insights from Predictive analytics might be:
- Product A and B tend to be bought together
- If a customer buys products C and D then it is extremely likely they will buy product E
- Multiple purchases with category W are common, and customers who make such purchases also tend to buy Y
Armed with this insight, retailers can make calculated decisions about how to match offers with customers. Retailers can predict who is most likely to participate and they can forecast the ROI associated with customer response. By applying Market Basket Analysis retailers achieve higher sales, greater returns on marketing spends and a more targeted approach to future offerings. At the same time, customers feel that their needs are understood and met. The payoff? Higher customer satisfaction and increased spend.
Data-Driven Decisions Deliver Better Results
Putting insight into action is critical. A retailer who can spot trends quickly and leverage them into operational and retail initiatives can differentiate their position in the market place. These patterns and insights also support decisions within the organization: how to differentiate assortment, plan merchandizing and manage marketing strategy and tactics to determine combined offers of multiple products.
Applying Predictive Analytics
Applying predictive analytics allows retailers to select the best models, delivery and communication methods and match them to customer profiles. For instance, a retailer may choose to send loyalty card holders personalized coupons with their monthly statement, send an offer to a customer while they are shopping online or suggest customers who bought product A might also buy product B. With predictive analytics driving these decisions, recommendations are accurate, reliable, repeatable and transparent. This ensures a high level of opportunity for up sell and cross sell, plus increased revenue per shopping basket per customer.
When retailers drive these models into a real-time environment, they can direct an online shopper to items of interest, saving time and increasing the likelihood of purchase. In these interactions retailers use knowledge of the customer’s profile to determine items that offer opportunities for upsell and cross sell. These models run in the background and can be deployed in real-time based on self-determined rules. For example, customers with good credit scores can be given credit whereas customers with undesirable credit, will be prompted to pay direct.
Your Analytics Journey
The first stage of analytics identifies who the customers are, segments them and reveals the triggers that encourage purchase. Taking what has happened in the past and applying it to propensity for purchase, service, or other actions the consumer may take in the future and then applying it in a real-time environment.
A major European department store analyzed sales and customer data to develop customer profiles, allowing them to accurately map preferences and use this information to improve operational and strategic initiatives. They recently launched a customer cross selling program using the monthly invoice where they could deliver a personalized proposal for each customer. Using predictive analytics they are able to make suggestions to the customer and deliver high conversion rates. This organization is also using predictive analytics to determine which advertising campaigns should be communicated to loyalty card holders — by focusing on the most likely candidates they saved an average of 30% on print runs for communications, while keeping the same turnaround metrics.
The Maturity Model
Want to utilize analytics throughout the organization? The business maturity model for predictive analytics provides a useful way to determine which stage you are at and what your next steps could be to leverage predictive analytics.
- At the Ad Hoc level, retailers typically use spreadsheets to do business planning. This is seen in command-and-control environments where decision-making resides in silos.
- At the Foundational level, retailers may be using data warehouses. There’s a governing structure for data, accessible to some but not to all in the organization. Teams tap into a reporting tool, and a typical implementation at this level would be task integration.
- When retailers reach the Competitive level, there is widespread use of dashboards and scorecards. This is where process automation and workflow come into the picture.
- At the Differentiating level, users have the ability to make predictions about the marketplace and to use business rules and patterns. CRM drives questions such as: Who is the best target for this coupon? Who should get this offer? What customer is most likely to defect and when?
- At the Breakaway level, this information-based decisionmaking is practiced among those at the top of the Retail Business Maturity Model. It is driven by fast analytics. It is prescriptive, real time and pattern based.
This maturity model can also act as a tool to help you discuss applications and broaden the usage of analytics internally with your colleagues, peers and staff.
A Smarter Merchandising & Supply Chain
Understanding consumer buying patterns is one thing. Having the right merchandising & supply chain to meet consumer demands is what really sets a retailer apart. This requires a “demand-driven” supply chain robust enough to eliminate store operational issues, such as delayed shipments, excess stock — or worse — empty shelves.
Analytics gives retailers complete visibility across the supply chain. Managers can identify key metrics and be notified when performance is off-track in order to make immediate corrections. Future results also become more predictable. Alerts identify conditions that typically lead to problems, enabling staff to take pre-emptive action to prevent inventory shortfalls, shipment delays and other events that hurt performance.
Building Smarter Operations
Today’s consumer-driven marketplace demands retailers that are lean and nimble. This means swapping out inefficient processes in favor of new approaches capable of driving optimal performance. Rather than setting targets at specific numbers, decision-makers may want to tie performance to events and risk factors. Rather than planning on an annual basis, retailers may consider adopting shorter planning cycles focused on achieving specific goals.
Through real-time information visibility, analytics empowers decision-makers to evaluate current and historical performance, forecast the impact of changes on planning, then implement action plans that best deliver anticipated outcomes.
Conclusion
In conclusion, shoppers rule and they are smarter and more informed than ever, so retailers hoping to succeed must keep pace. That means consolidating infrastructure to provide accurate information and consistent product availability, embracing the shopping channels consumers prefer and shifting to a more customer centric approach. This increases loyalty, retention and revenue on a per customer basis. To ensure success, retailers need deep insight into customer behavior, preferences and product needs. Predictive analytics allows organizations to leverage insights and apply practical usage across marketing and customer experience management functions for true competitive advantage.
Want more like this?
Want more like this?
Insight delivered to your inbox
Keep up to date with our free email. Hand picked whitepapers and posts from our blog, as well as exclusive videos and webinar invitations keep our Users one step ahead.
By clicking 'SIGN UP', you agree to our Terms of Use and Privacy Policy
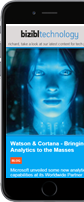
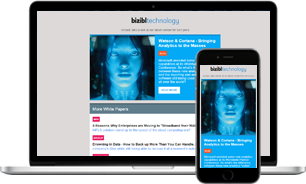
By clicking 'SIGN UP', you agree to our Terms of Use and Privacy Policy