Data Quality Management Method
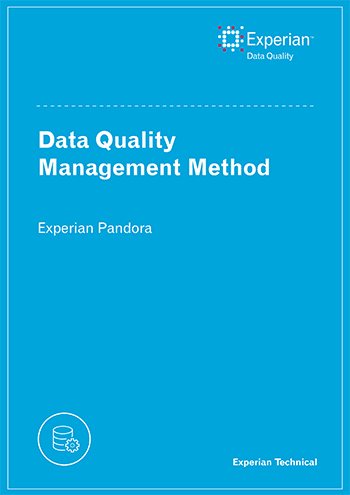
This document explains how to set up a successful Data Quality Management (DQM) process, outlining best practices in this field and how Experian Pandora supports them. It will allow you to justify and prioritise your data quality work.
Approximately half of businesses lose money due to data quality issues; unfortunately, most find that the exact amount lost is difficult to determine, and regard their data quality teams as cost-centres inflicted on them by over-zealous legislators and statistic-obsessed managers rather than potential profit-centres.
Experian Pandora provides a proven solution which associates business-defined relevance to its findings, producing financial, quantitative and directional Data Quality KPIs (Key Performance Indicators) backed up by all the detail necessary for correction.
Get the downloadBelow is an excerpt of "Data Quality Management Method". To get your free download, and unlimited access to the whole of bizibl.com, simply log in or join free. |
![]() |
1. Introduction
This document explains how to set up a successful Data Quality Management (DQM) process, outlining best practices in this field and how Experian Pandora supports them. It will allow you to justify and prioritise your data quality work.
Experian Pandora provides a proven solution which associates business-defined relevance to its findings, producing financial, quantitative and directional Data Quality KPIs (Key Performance Indicators) backed up by all the detail necessary for correction.
Common-sense should always prevail; you may find it appropriate to modify the suggested approaches for your particular organisation or project.
1.1 Data issues, so what?
Approximately half of businesses lose money due to data quality issues; unfortunately, most find that the exact amount lost is difficult to determine, and regard their data quality teams as cost-centres inflicted on them by over-zealous legislators and statistic-obsessed managers rather than potential profit-centres.
Some operational inefficiency, such as sending duplicate or incorrectly addressed letters, are costly but not usually business critical. The real cost of poor quality data is much higher however. Issues such as unsent invoices, payments for goods never received, deliveries of the wrong product, overstocking, stranded assets and mis-reported results are often the result of poor data quality, with potential costs (operational inefficiencies, fines and falls in share price) being measured in millions.
Few companies admit that poor data quality has cost 10s of millions in potential revenue, and more often than not the decision makers are unaware of it.
An energy company estimated lost revenues of £180M when data quality problems caused a project to fail, degrading relationships with customers.
Some companies attempt to understand and manage their data assets, often encouraged to do so by regulatory requirements, but we continue to hear about failures, and fines being imposed.
A water company was fined £26M by regulators for trying to cover up data quality issues created by a data migration project.
According to TDWI, only four out of ten people believe data quality improvement projects can provide a return on investment versus potential costs and fines.
A bank was fined $80M for not having an effective compliance process.
Data Quality initiatives are clearly not delivering on their promises, being technically complex, costly and slow to implement.
They sometimes produce colourful statistics and graphs, yet lack business relevance.
A Business Unit manager was told his system had 620 incorrect customer records.
He said, “so what?”
1.2 Your Data Quality Process
Start with a clear idea of the motivation and the boundaries of the process.
Activities should be dictated by the need to demonstrate a return on investment.
If there is a specific objective, then use it to give the project a name; “Purchasing Optimisation Project” is a much better project name than “Vendor Information Data Quality Project”….
1.3 Deliverables
The deliverables of the implementation of the Data Quality process are:
- Regular Data Quality reporting, with trending
- A Glossary of Business Terms, defined and associated with their actual data
- Re-useable “Content” – functions, patterns, domain files
- “Cleansed” data – depending on the project
1.4 Experian Software
This document uses Experian Pandora to implement the activities described, however the principles and approach can be considered best practice in the field of Data Quality Management and are applicable whether Pandora is used or not.
1.5 Experian Pandora
Pandora provides Data Quality Management functionality which enables organisations to:
- Identify, investigate and catalogue relevant data
- Assess and measure the value of issues
- Improve data quality
- Control this process over time
It does this independent of the data volumes, the provenance of the data or whether the information concerns customers, products, finance, sales or other business areas.
Experian Pandora will allow the relevant players in your organisation to quickly and easily provide a robust, flexible and transparent data quality management solution.
1.5 For More
For more information on how Experian Software could be helping your organisation, please contact us on 0800 197 7920, or send an email to dataquality@ Experian.com.
2. Tasks overview
This document describes how to set up and operate a Data Quality Management process.
The setup of the process requires initial activities to investigate, assess and improve your data.
Initiate
- Justify
- Plan
- Determine data sources
- Perform initial Data Profiling
Investigate
- Look for potential issues
- Improve the scope
- Document and prioritise the issues
- Document the non-issues
- Estimate complexity
- Start building a cross-system data inventory
Assess
- Plan
- Carry out detailed analysis and assessment (measurement)
- Create validation drilldown reports
- Complete the documentation of issues and priorities
- Create reusable content
- Improve Glossary Definitions
Improve
- Plan
- Find root-cause
- Correct existing data and prevent future issues
- Complete the documentation of issues
- Improve reusable content
- Improve Glossary Definitions
Finally you set up the continuous “business as usual” process which will control the data quality, and then loop back through the activities, investigate, assess, improve and control again.
Control
- Develop and save Content
- Develop “measures” and establish objectives
- Apply rules and Report findings
- Automate
- Take Action
- Perform Ad-hoc investigations
3. Some common-sense reminders
3.1 Get information from source
Where possible, use all the original source data for analysis, and analyse each source table of file individually. Sampling, or the use of previously developed extractions which were designed and built with other requirements in mind, often combining different source files and tables is always a source of error.
3.2 Involve the right people, the right way
IT and business, together.
The most effective way of working is to meet round a large screen and look at the actual data in a joint workshop. Of course an IT person can perform preliminary investigations to give data workshops some initial direction and questions to resolve, but a large number of issues are uncovered simply by interactively browsing through the data. Do not underestimate the enormous synergy generated by working together in this way.
3.3 Focus
Don’t get caught up analysing what appears to be the most interesting data from a technical point of view, analyse things that can have the greatest monetary impact on your organisation. The most frequent or amusing data issues are not necessarily the most important ones. One credit-card number embedded in a mailing address could cost you much more (in fines and lost reputation) than 100 inconsistent product descriptions.
When establishing priorities, also think about data relationships; missing records or records with the same key may not contain bad data, it is their presence, or absence which is the problem. For example, could it be very bad if a transaction from the sales system failed to reach the accounts payable system, or if a procurement item was paid for twice?
3.4 Follow the process
Stick to the documented sequence of tasks. When you start looking at data you can very easily get distracted and find yourself trying to build fixes immediately. If you have some ideas, note them and move on; don’t try to do it all immediately.
3.5 Manage data cleansing effort
In reality, businesses usually have to compromise on quality improvement goals in order to stay within the agreed budgets and timescales. If there are specific quality objectives for the project they should be clearly identified and their potential benefit quantified at the start of the project, allowing you to allocate an appropriate amount of effort.
Data Quality issues should be documented, categorised as either technical or business and fed to the Correction team so that the business can:
1. Evaluate the cost of repair vs. the benefit/risk to the business.
2. Prioritise the issues and assign an appropriate amount of resource.
The effort spent performing complex cleansing operations such as de-duplication should be related to their technical necessity and their benefit to the business.
3.6 Demonstrate Value
One problem with data quality initiatives historically is their lack of business-relevance.
Make sure that you know the value of the issues you are addressing, and measure periodic progress in terms of value rather than the number/percentage of records right/wrong.
3.6 Accept “good enough” Data Quality
We all want perfect data, but maybe 99% is “good enough” for your business, and the cost and time associated with correcting the last 1% is not justified in this business context.
A good example of this pragmatic approach comes from the Solvency II regulations for insurance industry. They state that insurers do not have to correct data issues, they simply have to know about them, evaluate them and take them into account in their risk calculations; the business people decide, based on their risk calculations, whether to fix the issues.
3.7 Use tools
Although you can do much of this with a combination of manual activity and software, try to use a tool which will support your process, from investigation and discovery, through prioritisation, development and implementation of rules, to presentation of the scores and “audit” of the whole process. The tool should allow you to work as a team, even if you are separated geographically.
Whatever your chosen tool, make sure you can get answers quickly; if it takes you too long to get answers to questions, people stop asking questions.
3.8 Be agile
You can’t plan for things don’t know about (yet).
It could be that investigations uncover an issue which should take priority over all you planned to do; so be objective, and be prepared to bin all your plans in order to address what you find!
4. Initiate
This document explains how to set up a successful Data Quality Management (DQM) process, outlining best practices in this field and how Experian Pandora supports them. It will allow you to justify and prioritise your data quality work.
Experian Pandora provides a proven solution which associates business-defined relevance to its findings, producing financial, quantitative and directional Data Quality KPIs (Key Performance Indicators) backed up by all the detail necessary for correction.
Common-sense should always prevail; you may find it appropriate to modify the suggested approaches for your particular organisation or project.
4.1 Objective
Determine the objectives, constraints and scope of the program, initially and going forward. Also determine who’s paying!
4.2 Inputs
4.2.1 Interviews
Watch out for end-of-year periods where people won’t have time to talk to you, and holiday periods.
4.2.2 Existing documentation
Where is it? Do you have the most recent version?
4.2.3 Data
Where is the data and how are you going to access it? Do you need to get permission? Does someone need to give you access. Get answers to this as soon as possible or it will delay your project
4.3 Deliverables
4.3.1 Project Justification Document
Explain why you are doing this.
4.3.2 Systems overview
An overview description & diagram of the systems involved and their relationships.
4.3.3 Scope Document
Establish the probable perimeter of the data investigations. This document lists the relevant data and where it resides. It is also useful to identify and explain any out-of-scope data that you have investigated.
4.4.4 Project plan
Now that you know which systems and files are involved you can construct an initial project plan. Include constraints.
4.5 Steps
This phase is based on workshops and interviews, as well as researching existing documentation. Both application experts and business people will be involved as we want to be sure we don’t miss any potential sources. It’s easier to exclude a source based on an investigation than it is to integrate a new source later on!
4.1 Justify
Establish why you are doing this work.
The motives of your projects will determine their type and therefore the approach used. We encounter four common motives for doing something about Data Quality (details below).
A good approach appears to be to use a specific objective to justify a project, and to take the opportunity to do some further rummaging around in your data once you’ve got your hands on it! Experian Pandora it will even proactively give you information about your data and highlight things which are statistically unusual without you having to actively look for things.
However you approach things you will always have to determine the project objectives, priorities, scope, constraints, timelines and sponsors/decisionmakers.
4.5.2 Plan
Objective projects
The project should finish once the objective is achieved, and the plan is based on achieving that objective. Although this is the easiest way to justify a project, it is clear that the work is seen more as a constraint or cost rather than as an opportunity to find efficiencies and added value. With the right approach, tools and attitude however you can use these projects to justify further work. E.g. Experience of Basel II banking regulations has shown banks that data quality can be used to competitive advantage.
- Go fix problem “X”
- Are we paying invoices for goods we haven’t received?
Motive 2. Another cost center
- Do something to keep the regulators happy
- My boss is asking how good our data is…
Subjective projects
The project will finish once the agreed resources have been used, so the plan is based on the use of the resources rather than any particular business objective.
Because of the lack of a clearly identifiable business objective this type of approach breaks the traditional rules of project planning. Companies are discovering however that such speculative projects, executed with rigour, almost always bear fruit, though not necessarily in the way anticipated. There is a significant increase in this type of project, which is based on “business hunches”. For these projects to be feasible, an easily accessible, agile approach is necessary.
Motive 3. Vague question
Someone in your organisation, it could even be you, is interested in data quality.
- Do we have any data quality issues?
- Is our data any good?
- It would be good to know why…?
Motive 4. Speculative/hopeful investigation
- Can we make savings from better data quality?
- Can we improve our sales by cleaning our data?
- If our data was better, more complete, more accurate could we make better decisions?
- In other words, can we gain competitive advantage through the use of our data?
4.5.3 Determine data sources
Build an overview description & diagram (e.g. Powerpoint) of the systems involved. Document how they should relate as well as the flow of data through the business departments and associated application systems.
Include decisions taken so far, e.g. to exclude certain subjects, systems.
Perform workshops with subject matter experts, profiling, viewing and manipulating source and target (if available) data to ensure you are talking about the same things and to get an early view of the data which the project actually has to deal with as opposed to what people think it has to deal with…
For Time-boxed projects
Identify the data which is the most important to your objectives, and work through it, top down, in priority order.
For Objective-driven projects
The scope is determined by the objective, however you will probably also need to prioritise your work and make compromises with some of the “nice to have” objectives.
You should determine which systems and then which tables/files to include.
Then, look around for other, unexpected places which may contain relevant data.
E.g. if you are interested in Products, then
- look for product identifiers or descriptions in places other than where you expect to find them (search through data values and patterns across all systems)
- find out which systems are related to transaction records containing products (relationship analysis)
4.5.4 Initial Access & Data Profiling
Initial analysis using Pandora to ensure you can actually access the data and that you have the relevant permissions, followed by brief work to validate and clarify the understanding of the data.
Preview and/or profile the data to evaluate:
- whether certain files and tables contain what you expect them to contain
- a cursory look at the quality of the data (are there values, do they “look alright”)
- are there records missing? If so you may need to look in places you thought were out of scope.
Concentrate on finding the most critical data and the information required to relate the in-scope systems together (if the investigation involves more than one system).
4.6 Tips & Techniques
4.6.1 Workshop
Always include IT and business colleagues during the scoping. A round-table discussion or workshop will provide the best results quickly.
If there is more than one potential source for some data, they should be identified now.
5. Investigate
5.1 Objective
Get an initial understanding of the data quality issues and their potential impact to allow prioritisation and planning of more detailed investigations.
5.2 Inputs
- Your data
- Existing documentation
- Information from interviews with subject matter experts
5.3 Deliverables
Notes for the potential issues
- Prioritised
- With a category
- With attached evidence
Revised Scope & Plan
- Revise the project scope and plan accordingly.
Glossary of terms
- definitions
- associated columns
- references to other documentation across your enterprise
5.4 Steps
Look for potential issues
There are three types of data issue:
- The bad data you know about.
Some of these issues are well understood, but many are “urban legend” and may not even exist. As you investigate you will understand the scale of the problems, and the knock-on effects they are having elsewhere.
- The unexpected data that’s not actually wrong.
Examples of this include Data fields being reused or unexpected values (a company which only delivers to its domestic market but which has customer addresses in another country).
- The bad data you didn’t know about!
This is the worst, because it wasn’t planned for at all, and may already be having a very significant impact on the performance of your organisation.
When you instruct Pandora to read your data it automatically profiles (analyses) all of it, proactively producing over 170 statistics for every column, and analysing all relationships and data overlap with respect to all data that has previously been analysed.
Data Profiling is about fully understanding data in order to make informed decisions about how it can be used and improved.
It is imperative that Data Profiling is performed against real data and that the data is as close to the real sources as possibly – ideally, extracted from the source systems as-is. Try to avoid using data provided by existing or custom-made extraction programs which filter, join, aggregate, transform or cleanse source data. Using data samples, old data or generated data may either obscure data problems, or mislead analysts into thinking there are problems there that do not actually exist.
You can then carry out three levels of analysis:
1. Browsing the automatically prepared statistics
2. Interactively manipulating data and metadata
3. Creating and applying specific rules
Use the Columns summary drilldowns and the Outliers report to start off this process by easily identifying unusual data.
Study the derived information to evaluate its quality
- Values and their frequency
- Empty fields
- Types of data
- Formats, and their frequency
Pandora lets you sort, filter, group and count to assist this process. The order and combination of resources and techniques you use to find issues will depend on factors such as your level of experience, how technical you are, and whether you are being driven by the investigation of a particular issue. The techniques for finding different types of issues are summarised below in the section Tips & TechniquesTips & Techniques.
It’s difficult to say how much time you should spend on these initial investigations, however if you average more than 15 minutes per column then you are probably doing more than an initial analysis.
Collaborate whenever possible.
- Carry out joint workshops with subject matter experts; looking at, manipulating and discussing the real data on-screen is extremely productive and can cut out days of email communication or discussions based on descriptions and expectations.
- Use the built in Pandora Chat to hold quick online meetings with colleagues.
Traditional Data Quality projects usually consisted of “validating expectations”, and occasionally being lucky enough to find unexpected issues. The tools available to project teams simply did not permit more. A benefit of using Experian Pandora is that it allows you to automatically find the unusual and unexpected data as well as discovering inconsistencies across tables and systems.
5.4.1 Improve the scope
Having looked at the actual data and carried out interviews you may uncover data in unexpected places.
If it appears that only a subset of some data is required, this should be documented now, and the criteria for selecting the appropriate records drafted.
5.4.2 Document the issues
As you investigate you will uncover “unexpected stuff”; the data may be wrong, it may be okay, you may not be sure if it’s okay.
Note
Create a note about what you find, include relevant associated information, then move on:
1. A description of what you think is wrong and why.
2. Information that will allow the importance of issues to be evaluated with respect to other issues.
3. Hints about fruitful avenues of investigation, and avenues of investigation which can already be ruled out thanks to your knowledge and initial investigation. This will save time when you or a colleague come back to do detailed analysis of the issue.
The amount of time you spend obtaining this information depends on its relative priority. Remember, if you spend too much time on investigation now you may fail to reach the even bigger issue waiting for you in the next column….!
Notes should be categorised and have an “importance” assigned to them so that they can be used to prioritise future work.
Evidence
Attach evidence to notes, in the form of drilldowns of data and metadata, allowing you to quickly answer the two questions you are always asked when you tell a colleague about an issue:
- Do you have a specific example?
- Can you quickly give me an extra piece of information which is elsewhere on the record or in another related file?
Workflow
Assign notes to colleagues to allow you to
- ensure important work is not forgotten
- retain focus on the task at hand.
Audit & report
The “bulletin board” style of Pandora notes allows them to serve as an “audit trail” of project activity:
- who said/decided what, when
- prove diligence in your analysis process to regulators!
Furthermore, the search and export facilities of notes allow you to use them as simple project reporting. E.g. search for and export all “conclusions” to an HTML document.
5.4.3 Estimate complexity
An initial evaluation should be made of the complexity of the data to assist estimations for the project plan. This can be as simple as “difficult, medium, easy”, because even that is useful. As your gain experience in this process you will be able to refine your estimating into something a bit more accurate.
5.4.4 Document the non-issues
If you find some data which looks suspicious, then on closer inspection you realise it’s okay, create a note to say so. This will avoid your colleagues (and you) re-analysing the same suspicious data at a later date.
5.4.4 Start building a cross-system data inventory
As you investigate your data, take advantage of the fact you’re there to associate columns and fields with the relevant Glossary terms.
This allows you to associate the same information which exists in different locations, information vital for any project hoping to subsequently use the data:
- Is there data duplication?
- Are formats consistent?
- How many different sources need to be coordinated and consolidated?
If there is no appropriate Glossary term yet for the data you are looking at, create a candidate definition yourself or ask the data steward to do so.
Types of information you can store as part of your definitions are:
- Description, format, examples
- Synonyms
- Data custodian (steward – the person who represents the data owner)
- Data owners – not the same as custodian. This person is responsible for making decisions about the data. This may well be the person the regulators consider to be legally responsible for the data.
- Where and how is it stored, archived and backed up
As always, fill in what you know, and move on, creating notes on the Glossary terms and possibly assigning them to colleagues so that the activity can be continued at a later date or by someone else.
5.5 Tips & Techniques
5.5.1 Broken process (relationships)
Relationship analysis is able to highlight issues in the data created by incorrect or fraudulent activity in application systems. Broken data is the “smoking gun” with respect to broken processes.
- All relationships are discovered automatically by Pandora
- Chose the tables columns to view using the relationship finder
5.5.2 Consistency
Conflicting Datatypes are easily visible in the Columns summary drilldown.
Dependency Analysis will automatically discover inconsistencies based on precise statistical analysis of all data.
5.5.3 Completeness
A completeness percentage is included automatically on the columns summary.
The Explorer allows you to view all rows with missing (null) values, and there is a right-click option on the columns summary to get to the same information.
5.5.4 Combinations of Values
Interactively Group the data by multiple columns and apply count/sum functions to find unusual combinations of values. Useful aggregations are count, sum, distinct group values (obtain the list of unique values present in a column for each group)
5.5.5 Uniqueness
A uniqueness percentage is provided on the columns summary; a uniqueness of almost 100% is suspicious. From the Explorer you can view all values and their frequencies.
5.5.6 Validity
Compare the actual values with the list of valid values. You will use lookup rules and domain tables to automate this later.
Study the information on the columns summary drilldown, such as low/high values and the number of formats.
Study the Profile information in the Explorer to uncover any rare value formats.
5.5.7 Conformity
Compare the actual data formats with what you know to be the valid formats.
5.5.8 Finding the same type of data, elsewhere
Look for attributes that have similar names. Pandora allows you to view, sort and filter all field names.
Attribute names may be similar though not identical, yet have similarly structured data. For example Product Id and Product Code. So look for values with the same format using the right-click options on drilldowns.
Look for columns which have common values – use the relationship analysis.
5.5.9 Mis-placed/Hidden data
Click right on values or columns to find other columns containing some of the same values or containing values with the same format.
Use relationship analysis of a single column to find all other columns which have values in common.
See unexpected values on the columns summary drilldown (minimum, maximum, longest value, etc.)
Use filters and expressions to search through all values (by table or globally) to find embedded values (by value, by format, by sound, and including well known “aliases” thanks to Pandora “domains”)
Use a pattern (regular expression) to find bank account numbers or credit card numbers embedded in free-text values.
5.5.10 Keys
Use key analysis to validate your expectation that the values in a particular column or a combination of values in more than one column are unique.
Use Pandora key analysis to automatically discover all potential multi-column keys.
5.5.11 Integrity
Use relationship analysis between tables and files.
Add expression columns which perform (multicolumn) lookups to other tables to validate the presence of associated records.
5.5.12 Outliers
Use the Outliers report to view and investigate data which is statistically unusual (based on “standard deviations”).
- Split screen and investigate each one
- Document findings as you go
5.5.13 Compliance/Audit/Fraud investigation
Use Pandora Context-free, quick-search to find any records containing some combination of the words you supply – uses multi-string fuzzy searches.
Perform pattern and value searches across single tables and across all tables
- e.g. look for credit card numbers, phone numbers or profanity.
5.5.14 Transactional Data
Transactions produce high volumes of data. Records are typically short and the data is highly repetitive. Usually, transactions contain references to “reference” or “master” data, rather than containing those types of information. Because of the nature of this data, if you analyse a few hundred million records from a single table you can consider that to be “representative” of all the data in that table, and you are likely to find all the table’s issues within that sample. If you want to be 100% sure, you can profile up to 2 billion records from a single table with Pandora. If you need to analyse more, set Pandora load parameters to analyse the data in “chunks” of 2 Billion at a time.
5.5.15 Subsets
Work on subsets of data by interactively filtering the data on-screen:
- Use the Drilldown manager (filter funnel icon on drilldown toolbar)
- Right-click filter data and include/exclude rows
Once the data has been filtered, use interactive oneclick profiling of the data.
5.5.16 Duplicates
The standard profile provides value frequency information showing all values that are duplicated.
Identify duplicates using the quick, simple interactive technique of transforming/ standardising values (e.g. remove noise), and then studying the value frequency of the standardised values. E.g. Right-click from drilldown, Group, then Distinct Count to see multiple surnames for a telephone number
Relationship analysis will find duplicates across multiple source tables, because they will show up as common values.
6. Assess
6.1 Objective
Get a detailed, measured understanding of the data quality issues, their priority and their potential impact to the business, allowing prioritisation, justification and planning of subsequent data improvement activities.
6.2 Inputs
- Your data
- Pandora Notes
- Glossary Definitions
- Information from interviews
6.3 Deliverables
6.3.1 Completed Notes about issues
Additional notes and/or additional details with more attached evidence.
6.3.2 Improved Glossary definitions
Definitions should now be “approved” rather than “candidate”.
6.3.3 Validation drilldown reports
Allowing “regression testing” of your data quality.
6.4 Steps
6.4.1 Plan
By this point you will have a list of issues from your investigations. You should now pull together subject matter experts and business people to review and prioritise these issues. Once prioritised, work out a plan based on your resources and available time. Depending on what has been uncovered, and what you discover in this phase, you may need to obtain more resources (or less!) as you progress, but of course you are doing this based on evidence and priorities which have been objectively set, rather than speculation, so it should be a (relatively) simple business decision.
Be agile, and change the plan when required if you uncover more important issues. Thanks to “notes” you can easily come back to some unfinished analysis at a later date.
6.4.2 Carry out detailed analysis and assessment
Start with the evidence collected during the earlier investigations (notes & attachments) and quantify the issues.
Specify and prototype rules to measure quality:
- Create & validate the prototype rule in a new drilldown column
- Use the 300+ Expression Editor functions as building blocks to combine and transform data as part of the rule
- Output is a true/false result (for validation/ measurement)
Analyse relationships as well as the content in each column.
e.g. There is a relationship to customer telephone number from the telephone number table. Whether customers have one or many telephone number records, or have any numbers at all will have a fundamental effect on any processing that use this data, so you need to understand the actual relationships.
Work with business people and try to establish a relative financial value for issues, based on a monetary/risk value (premium amount or insured amount). The value can be based on an amount in another column of the same table, or calculated/ derived dynamically using rules. You can also use reference data from other systems & Domain files.
Include external domains for recognition, validation, translation and standardisation.
6.4.3 Create Validation Drilldown reports
You will develop data quality measurement rules in drilldown windows. Use the “drilldown summary” to create quick reporting to communicate with colleagues.
It is often the case that data fixes can be quickly made by the business. By saving the drilldowns which contain your “in progress” validation rules you provide a quick method of evaluating those fixes when the data is refreshed (re-loaded into Pandora), because Pandora automatically copies all such drilldowns to each new version of the data.
6.4.4 Complete the documentation of issues
Add Details to the existing Notes, associating more evidence each time.
6.4.5 Create reusable content
Create & share experience
- Save Validation Function to the Glossary ready to apply to a table for ongoing monitoring later
- Create and save your own reference data (lists, aliases, patterns)
6.4.6 Improve Glossary Definitions
Add to and improve the definitions according to the detailed investigations you have carried out. Make reference to your sources of information and where they are stored.
6.5 Tips & Techniques
6.5.1 Work with subsets of data
Identify subsets of data within the same data sources (tables) and create named filters for them (table filters). These can be created to exclude certain records, or you could create filters for different logical subsets of the data within a single physical table.
e.g. data from different countries or for different product categories which are only stored in a single physical table for technical convenience.
You may even want to profile only a subset of the data, in which case there are three choices
- Create a View on the source system, so that only the relevant data is read from the source. Pandora treats this view as a table in its own right.
- Perform interactive one-click profiling of relevant columns in the drilldown window.
- Use “save as a table” to profile only the data that is part of your subset as if it were a source table in its own right.
A table may contain unwanted records (within the context of the current target) which you want to filter out, e.g. history records, inactive records, unwanted record types or even duplicated records. Filters can be applied to the data at any time using the Pandora interface.
6.5.2 Duplicates
Take a few seconds to apply standardisation/ remove noise rules to text fields such as product names, telephone number or customers, then “right-click profile” the result. This is a simple fuzzymatching technique which finds duplicates. Use more sophisticated rule-based standardisation to significantly improve identification of duplicates, e.g. using domains and aliases, pattern-based parsing and removal/replacement. This activity should be “time-boxed” according to its potential value. An experienced Pandora user can find “most” duplicates in a table with about a day of work.
6.5.3 Missing records
Finding missing records is far more difficult than finding duplicated values. The techniques rely on finding broken relationships.
Sequential keys
Look for columns containing sequential numbers where there is a missing number, e.g. 1, 2, 3, 4, 6, 7, … . The outliers report highlights this type of problem.
Previous/Next record comparison
Create new columns which use the “cell” functions to look ahead and back into other records. By comparing values you can find unexpected differences.
Relationship Analysis across systems
If a transaction identifier exists in one system but not in another related system, then this will show up as a relationship with less than perfect quality.
6.5.4 Relationship Analysis
Load the identified sources into Pandora, and all relationships will be discovered automatically based on common data values.
The relationship between two sources may be evaluated in Pandora at any one time to ensure that it is exactly as expected. If you are dealing with data from multiple systems or files you have no choice but to rely on Pandora to find and validate relationships because there is no “schema” or “constraints” between systems. Some systems have some or all of their “constraints” deactivated, preferring to let the applications manage the integrity of the database, and so once again Pandora is the only way to find these relationships (unless you want to read through all the code of all the programs in your applications!).
If you are not sure about the relationships, e.g. there are unknown “link” tables between the main data tables, start from what appears to be the main source table, then:
- Discover (find) the unique key(s) for that table
- Find all relationships with other tables which rely on the key field(s). Those with the best quality relationships are related!
- Continue out from those tables, analysing relationships to empirically discover the model
Multi-column keys
For multi-column keys, join on the most unique of the key columns, and use the drilldown manager (filter) to add the other columns to the join condition.
Non-identical keys
If you want to use a relationship which is not based on single unique values you may need to create a derived join key, and re-analyse the result, i.e. create a new column using the Pandora expression editor, and save (materialise) as a derived table.
Relationships can be optional or mandatory. Furthermore, they will be one-to-one, one-to many, or many-to-many.
Beware differences in levels of aggregation
Be wary of relationships which are based on a setbased transformation of a source table, e.g. join to the most recent delivery record, or join to a total, by product of the sales records. These may well require you to save some kind of aggregated/transformed table to avoid ending up with duplicated records in your applications.
7. Improve
7.1 Objective
Decide how to improve the quality of the data, both now and in the future.
7.2 Inputs
- Pandora Notes
- Your data
- Subject matter expertise
7.3 Deliverables
7.3.1 Validated data transformation design (rules)
A generated HTML document showing pseudo code/function calls to enable implementation
7.3.2 Fully/partially corrected data
Data which has been transformed using Pandora expressions and exported.
7.3.3 Detailed lists of data errors
Exported data which has failed the tests you set.
7.3.4 Notes containing suggested improvements to business processes/training
Help for future work.
7.3.5 Notes containing suggested ways to monitor the progress of data quality
Suggested rules for data quality monitoring.
7.4 Steps
You should consider improving data in two ways:
- Improve the data that exists
- Avoid future data quality issues
7.4.1 Plan
Use the previously established measures to determine next steps
- Justify action/inaction, e.g. For regulators!
- Set priorities for investigation/resolution
Chose a pragmatic approach according to the scale and importance of the problem e.g. Manual fix by a business team if only 20 cases to correct; carried out immediately if deemed necessary by the business.
Decide if, how and where to fix the data.
7.4.2 Find root-cause
Analyse incorrect data to identify root causes. Profile the bad data to find out what the records have in common. E.g. same business area, same product type, only occurs on certain days, …
7.4.3 Prevent future issues
Once you know where the data is going wrong you can investigate why and see if there is a fix.
Determine whether you are likely to see more bad data being produced in the future, and whether some action is required to avoid this:
- Often, better staff training will avoid people “getting round” the system. Data can be monitored to evaluate the effectiveness of the training…
- Sometimes, changes to the application systems or the business process itself prevent certain errors happening.
Compare the cost of prevention with the measured (potential) cost to justify any activities.
7.4.4 Correct existing data
Based on your analysis, decide how to address the issue.
There are many possibilities:
- Can the data be corrected at source? Even manual fixes are feasible if the volumes are small.
- Can you build a corrected version of the data
using Pandora and/or manual editing of a file? If so, this corrected version could be applied to the source by the people in charge of that application, or used as a “lookup” table by a data movement process – i.e. for this old value, replace it with this new value.
- Is it enough to simply “name and shame” the responsible areas and let them decide how important and urgent any potential fixes are.
- Is there any point correcting existing errors? Maybe preventing the issue at source is enough, and you do nothing with existing errors.
Whether you decide to correct the data or simply design the correction logic, Pandora provides over 300 cleansing and standardisation functions.
You should interactively build & validate data improvement rules as you did for the validation rules, however you are now constructing useful data rather than yes/no validation results. You will be doing the equivalent of “writing a specification” and “validating it”.
- Prototype rules to ensure they work (this is Agile for Data). Build rules incrementally, validating the results at each step using the actual data on the screen.
- Export corrected data and/or specification.
If Pandora is used to provide a list of “candidate” duplicates, the list can be manually refined and used as-is or as a “lookup” in the subsequent data de-duplication process.
7.4.5 Complete the documentation of issues
Add Details to the existing Notes, associating more evidence each time. At this stage you may be stating your approach to fixes and attaching drilldowns of data that you have chosen to send for manual correction etc.
If fixes are manual make sure there is some kind of audit trail, and create a note inside Pandora about the fix (who, when, where, approver etc.)
Improve reusable content
You will improve your reference data (domains), patterns and rules as you gain experience, and these improvements are then available for future use. Systems change, so your reference data may simply need additional values.
7.4.6 Improve Glossary Definitions
Add to and improve the definitions as your knowledge and practical experience of the data increases.
7.5 Tips & Techniques
7.5.1 Duplicates
If data needs to be consolidated/de-duplicated, potential “matching” information should be identified. Get a feel for how effective this consolidation will be by trying it out using functionality from the Pandora expression editor. For example, standardise data by removing all blanks and punctuation, changing all to upper case, then compare records based on this prepared “matching key”, or perform an interactive Grouping and Count aggregation on the derived values to see which are duplicated.
Take advice from subject matter experts.
- How do you know these records are for the same product/ person/ company etc.?
- Why is this particular product/person/company etc. more important than the others?
- What is the risk/cost if this type of information is not de-duplicated/consolidated?
- Separate “technical de-duplication” from “fuzzy-matching”. The former involves making allowances for technical differences such as upper/lower case, or data definition differences, e.g. a bank account number could be stored as a “string” or as a number in a system, making them different strictly speaking, even if they are the same in business meaning and content. The latter involves making allowances for material differences and usually relies on context-specific rules, fuzzy comparison functions and reference data.
“technical de-duplication”
It is possible at this stage to rely on some simple transformations such as changing case, removing spaces and punctuation, changing data-type and padding/formatting values, however you should not be tempted to modify/standardise/cleanse the values beyond these simple technical operations.
Use interactive Grouping and aggregation (count) on the values to see which are duplicated.
“fuzzy-matching”.
Move on to the more open-ended fuzzy matching activity which should be a time-limited activity. Use domains of values, aliases, regular expression parsing & replacement to standardise values and make them more easily comparable.
7.5.2 Standardisation
The Pandora parsing, pattern matching, string manipulation and domain functions are extremely powerful and ideally suited to this activity. Develop domains of values and patterns (using regular expressions) and use them to recognise, split out and standardise values. Comparison of records is much more effective with these standardised values.
Most companies can re-use existing reference files, and some Experian partners propose packaged solutions containing domains, formats, cleansing, standardisation and matching rules.
Translation tables are frequently used. These can be built using Pandora, and the implementation team can chose to either simply use the table (if the data is stable) or to program the rules applied by Pandora to build it.
A seasoned Pandora user can create an entire set of standardisation and cleansing rules and use them for matching purposes within a couple of days, including preparation of translation and reference domains and validation formats.
Common transformation include:
Datatype Translation
There is often the need for datatype conversion, e.g. from alphanumeric to numeric. This should be examined carefully using the type information in the Pandora profile.
Format Translation
Once the required format of the data is understood, the source field can be profiled to see how well the format matches, and appropriate transformation rules to rectify the format can then be built.
Semantic Translation
Data could potentially be of the right physical type but semantically incorrect/inconsistent for the system, requiring translation. A common example of this would be a coded field such as Gender which expects uses ‘M’ and ‘F’ to denote male and female, whereas some records use a coded system of ‘1’ for Male, ‘2’ for Female and ‘3’ for unknown.
7.5.3 Create Domain and translation tables
Another type of reference table often created is “alias” tables. These can be used both by Pandora and by other data management processes because they are contained in simple delimited files. Domain tables can be created and maintained by business people with no access to the Pandora software.
Example approach for building a domain table:
a) Group-by (combinations of) unique values
b) Apply a count to ensure they are unique
c) Filter out unwanted values
d) Fill in missing values from other columns or even other sources (using expressions and joins)
Domains can integrate any reference data (in-house or external)
8. Control
This activity represents the continuous business as usual process. It is part of what can be termed “operations”, however it is an activity which exists alongside the operational systems, providing independent, objective audit and control of the data in those systems.
You should consider broadening the scope of such ongoing monitoring to include “operational” information, such as access logs, file lists, security warnings,...).
8.1 Objective
Measure data quality over time, providing statistics and showing trends.
Enable periodic ad-hoc investigation of data to uncover new issues.
8.2 Inputs
- Data
- Existing rules
8.3 Deliverables
8.3.1 Statistics over time
Built up automatically, with trend lines shown.
8.3.2 Improved rules and reference tables
Logic and supporting reference data. Some of this can even be found on public web sites like Wikipedia.
8.3.3 New issues for investigation
A list of things to investigate, going back to the start of the process.
8.4 Steps
8.4.1 Develop and Save Content
Finish off and save the validation rules as reusable functions.
Develop and save reusable Business Constants
- Store constant values used by Rules (e.g. Tax %)
- Useful patterns (regular expressions), postal code, email address, driver number, ...
Create or purchase files of reference data (domains) – first names, valid postcodes, ISO codes, etc.
Finalise Business terms
8.4.2 Develop “measures”
You will already have defined many of these during the detailed assessment.
Finalise how you intend to associate objective measurements with your data quality. For each table or KPI (Key Performance Indicator), get the business people to define how they would put a value on the data which is relevant to your business and encapsulate this as a Pandora “measure”. E.g. high-value transactions are most important
8.4.3 Establish Objectives
Establish quality objectives. Maybe 99% is tolerable? Pandora allows red, amber green indicators for passed failed and tolerable.
8.4.4 Apply rules
Apply rules to the tables and columns you wish to measure.
From now on these will be copied and automatically applied to all more recent versions of the data. Many of the rules exist already from the Assess activity earlier and simply need to be applied. These rules can and should be improved over time.
- Set table thresholds – red, amber, green
- Work on a subset – use a table filter
- Add specific filter criteria to each rule
- Can be FYI only (not in consolidated results)
Pandora will automatically show reports with count passes/failures (Quantitative KPI), and trends over time (Directional KPI).
Pandora provides some built-in validation such as:
- Country codes
- ISIN validation
Since Pandora keeps all data, remember you can even add rules retroactively, thus resolving the age-old arguments about whether a just-discovered issue is truly “new” or “old” and had simply not been spotted before!
8.4.5 Report
Provide interested colleagues with the dashboards as well as supporting details using “notes”.
A Data Quality Dashboard is created for each table, each rule and each column.
Pandora keeps the statistics of all the validation rules meaning that you can show improvement!
It is also possible to report using global Data Quality Dimensions (Rule Groups), defined in the glossary and attributed to each rule. E.g Validity, completeness, integrity, …
Reports can include the following:
- Dashboard for each rule and for each table
- Real-time interactive drilldown to all underlying data (pass, fail, ignored, all)
- DQ dashboards added automatically to Data Quality Reports
8.4.6 Automate
These statistics should be set up to be refreshed on a regular basis.
Choose an automated process, time-based or trigger based to ensure no-one “forgets”, and to allow results which are consistently spaced over time.
These over-time statistics are considered to be directional DQ KPIs.
Ensure that you will have the necessary security to read the data on a regular basis.
8.4.7 Take Action
Drill to records in error, and analyse them (use interactive profiling).
Go through the usual improvement process
- use the already available information to prioritise and justify actions and improve the existing business and IT processes.
- Use one-click “profile” of this particular subset of the data to try to uncover root-cause.
- Sort the erroneous records according to their priority (measure), if there is one.
- If some high value records are wrong, it may be appropriate to send them straight to the business area for manual correction.
Improve the rules if appropriate.
8.4.8 Perform Ad-hoc investigations
This is like going back to investigation.
If what you uncover is interesting:
- Determine the data owner and how important the data is
- do a detailed assessment
- put a value on it
- find out where the problem came from
- decide if/how to prevent future problems at source and fix existing data
- fix
- include new rules in the ongoing monitoring
8.5 Tips & Techniques
8.5.1 Different ways of measuring the same data
For more sophisticated/flexible quality measurement, add rules to the columns (from Explorer) rather than the table. The statistics generated on columns are not consolidated up to the table but they are visible on demand.
Column rules do not impact the Validation scores. This is measurement only, e.g. Feasibility, “What if” scenarios, ...
You can weight results differently, for different objectives, and use different rules from those used in table validation (e.g. For different Departments or project teams).
9. Weighted Measurement
This chapter describes how to provide data quality measurements with relative weightings, and how to aggregate or summarise them in various ways.
9.1 Definitions:
9.1.1 Measure
- This determines the importance of each record
with respect to other records by weighting
them according to a monetary amount or a
risk.
This is implemented by choosing a column or “table expression” as the “measure” column for the table or column.
9.1.2 Weighting
- This determines the importance of each column with respect to other columns in the same “grouping”
- This is applied using the technique described here.
9.2 Weighting detailed results
1. Set rules on a table (or columns). If you need rules on the same table to have different measures, those rules need to be placed on columns.
2. Assign each rule to a “Rule group” (defined in the Glossary). If you need a rule to participate in more than one rule group it should be applied multiple times, each time assigned to a different rule group.
3. Bring up a drilldown containing the rules you want to weight and summarise. e.g. the rules for a Rule Group, or the rules for a table, or All Rules.
a. Add a new column to calculate a weight for each column (see technique for weighting below)
b. Add another column which shows the result of multiplying the score by the weighting
You now have detailed weighted scores, which you should save as a drilldown.
9.3 Technique for Weighting
1. Create a lookup table which uses 4 attributes as its key (Group, Table, Column, Rule Name) and has a column with the relative weight of the corresponding column for that Group.
The lookup function applied to the rules drilldown uses the values in the other columns of the drilldown (Group, Table, Column, Rule Name) as parameters to find the corresponding weighting record which is then used in later calculations.
The lookup table could be a CSV maintained outside Pandora, but in that case all uses (drilldowns) would need to be manually updated (novated) each time the table is refreshed (reloaded).
2. Lookup ALTERNATIVE
Use an “alias” domain table as a lookup table
The “alias” value is the concatenation of Group, Table, Column and Rule Name
The “standard” value is the “weight” amount.
This can be maintained outside Pandora and will be automatically refreshed as soon as the file is saved, so is a better solution then the first, though slightly less intuitive.
9.4 Avoiding Rule Groups
The use of “rule groups” is not necessary for weighting and summarising
If you view a drilldown of the list of all rules (rightclick on database and view all rules) you can use filtering to remove some rules leaving only those you wish to be considered together.
The drilldown itself is the definition of your “group”.
9.5 Overall Current DQ Score
1. View all rules (right-click on “database”), or latest versions of all rules.
2. Add columns to give the weighting and weighted scores (percentage and amount) as described previously.
3. Decide how you want to summarise the scores. By default you can use “Rule Group”. Right click and Group By the chosen column.
4. Filter out any rows (Groups/table versions) you don’t want included in the DQ average.
There are options which only display the most recent versions of all rules, so this may mean that you require no other filtering.
This screen shot shows two lists of rules in two sideby- side drilldowns, All, and All(Latest Version).
5. Now add your averages Click right on the column headers and apply aggregation functions
a. SUM the weights
b. SUM the scores
c. Create a new column by dividing the summed score by the summed weight.
You now have the weighted average for the rules, by Group.
Save this as a drilldown too.
6. If you want an average for your enterprise: Remove all grouping.
You could also add a column based on the names of the groups to give Groups a relative weighting before Averaging them.
7. Save these as drilldowns. To refresh them you simply need to change the “filters” to exclude all but the most recent versions.
5. Now add your averages Click right on the column headers and apply aggregation functions
[Download PDF to see Screenshot Example]
Want more like this?
Want more like this?
Insight delivered to your inbox
Keep up to date with our free email. Hand picked whitepapers and posts from our blog, as well as exclusive videos and webinar invitations keep our Users one step ahead.
By clicking 'SIGN UP', you agree to our Terms of Use and Privacy Policy
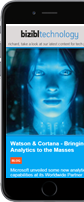
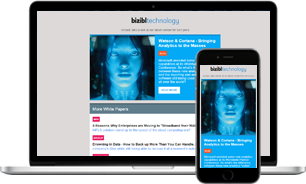
By clicking 'SIGN UP', you agree to our Terms of Use and Privacy Policy