Data Monetisation by Design: Five steps to plan for success
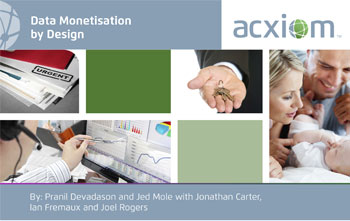
Marketers have been monetising data for years but today, fuelled by an explosion in data and driven by more ambitious brand strategies, marketing professionals need to think carefully and act confidently if they’re to achieve a competitive advantage and avoid losing out. It is possible to be opportunistic and monetise data tactically, but to be a long-term winner brands must embrace ‘data monetisation by design’. Read on for five practical steps to deliver that vision, a vision that can create very significant, sustainable revenue streams and a real competitive advantage.
Get the downloadBelow is an excerpt of "Data Monetisation by Design: Five steps to plan for success". To get your free download, and unlimited access to the whole of bizibl.com, simply log in or join free. |
![]() |
Executive summary
Marketers have been monetising data for years, but today, fuelled by an explosion in data and driven by more ambitious brand strategies, marketing professionals need to think carefully and act confidently if they’re to achieve a competitive advantage and avoid losing out. It is possible to be opportunistic and monetise data tactically, but to be a long-term winner brands must embrace ‘data monetisation by design’. A practical, structured plan should be followed to deliver that vision, blending short-term wins with long-term strategies that can create very significant, sustainable revenue streams and a real competitive advantage.
Introduction
‘Data monetisation’ as a term is a relatively new phenomenon. As recently as the last 12 months have Acxiom’s clients and partners started asking for guidance in this area and Google Trends only really registered data monetisation at the beginning of 2012 as a significant search term:
While the term may be new, the concept of data monetisation has been evolving without the moniker since the early days of the National Change of Address service offered by Royal Mail. The initial intention was to provide a mail-forwarding service to moving householders but marketers were quick to appreciate the predictive targeting value of knowing someone has moved home with the associated spike in expenditure on all things from DIY to household products, and potential switches in utilities and financial services. The same could be said of P&G’s Pampers programme with Bounty. Its prime raison d’être was to promote the sale of disposable nappies, but the data indicating a new child in the household was, for marketers, and therefore Bounty, something of great value as marketers again sought to understand and target the changing needs of the household.
For most businesses there are two obvious options to create incremental revenue:
- Better meet the needs of your customers to drive increased loyalty and customer value
- Help a third party better meet the needs of their customers
A simple model for data monetisation is ADA™: Application, Data and Access. Like the proverbial three-legged stool, each ‘leg’ needs to be present for data monetisation to work:
Application – for example, the ability to target a household based on a major life-stage event.
- Is the application credible? Why will companies want to buy the data product?
- How can the consumer be better targeted or served?
- Is it commercially viable; what will the ROI be?
Data – what data is required to fuel the data product?
- Does the data exist (Availability)? Are there 1’s and 0’s out there (or inside the organisation) that could enable the application?
- Is there enough of it and is it accurate (Integrity)? • Could the data be created/collected?
Access – can you get that data if it does exist? Is it commercially available?
- How will the data be received (real-time, batch or feed) (Practicality)?
- What permissions are associated with the data? Take care to not abuse the trust placed on you by the consumer to use the data they have shared with you responsibly (Privacy)
An instance of contemporary monetising of data by selling a service to a third party is Weve, a joint venture (JV) recently agreed by three mobile network operators. In addition to a combined mobile wallet, the JVs want to provide businesses with services based upon the data the networks collect as consumers go about their daily routine. The opportunities for location-based services are immense, especially when combined with the data trail that users leave behind accessing different websites and online services.
Data monetisation typically happens in one of three ways:
- Inside-In – where the marketer seeks out ‘lazy’ data assets within the organisation and applies them creatively to drive new revenues. (Where this is nothing more than doing a better job of data-driven marketing it should not be termed data monetisation, which should be reserved for the more creative applications of data to step-change revenue.)
- Outside-In – where the marketer seeks out third-party data to enable their strategies
- Inside-Out – where the marketer identifies powerful internal data that is of value to other marketers and brands
So is data monetisation really something new and exciting? Yes and no. For some businesses it is a new opportunity to open up revenue streams; but other organisations had been doing it for some years before data monetisation became popular terminology. Regardless, the timing of the increase in hype is easily traced and can be linked to the ubiquity of high-speed broadband, 3G networks and smartphones changing consumer behaviour in many ways, including media consumption and shopping habits. The resultant shift to digital has often left well-established businesses facing a decrease in profits as their customers expect to share in the financial benefits through passed-on discounts.
On the plus side, going digital means businesses are capturing more information about their customers than ever before, which in turn opens up new opportunities through using that data in other ways, including monetisation. This increase in consumer data is one of the key drivers behind the gathering storm that is ‘big data’. Numerous technology vendors have brought solutions to the market to allow businesses to analyse this big data. However, the benefits are less well defined; industry analysts recommend businesses invest in ‘data scientists’ to start interrogating these big data assets and make unexpected discoveries. Some commentators have felt compelled to ask “What is a data scientist?”; such is the mystique surrounding this new job role. Some businesses will find it difficult to decide whether the role is relevant to them. Hilary Mason of Bitly describes the data scientist job role as “someone who blends math, algorithms and an understanding of human behaviour with the ability to hack systems together to get answers to interesting human questions from data”; or, less poetically, as an “awesome nerd”.
Obviously this data analysis is only a means to an end, and data monetisation is a term that is being used to describe the end result, or one of them. It also has the advantage of having more appeal in
some boardrooms than trying to get approval for an entire big data project or hiring a team of data scientists which some infer as being more about the means than the end.
But, whatever the method, big data and data monetisation are linked, although both also have relevance in isolation. You don’t need big data in order to monetise your data asset, but it certainly helps.
Data Monetisation — Acxiom’s Point Of View
Our point of view is this: don’t let data monetisation just happen, instead pursue ‘data monetisation by design’. Knowledge is power and in marketing terms, data drives better results. With rapid and constant changes in consumer technology and behaviour driving big data, brands that plan to use data strategically will outperform those that do not — it really is that simple.
So what does data monetisation by design mean? It involves two key mantras that link directly to the ADA model:
- Put the consumer first
- Be opportunistic but plan strategically
Brands and marketers must accept that increased revenue almost always flows because a consumer has said ‘yes’; because they have seen value in your products or services or had a great experience. But to get to that point, the offer and experience has to reach that individual in the right way at the right time. Naturally, this is where the data plays its part. So it follows that marketers should first think about how they can better serve the consumer and work back to the data they need; this is the best way to guarantee any results through data monetisation. Of course, putting the consumer first also means protecting their interests — this is the Application of ADA; if it has added Credibility it will benefit the consumer. There may be bad players in the market but their misuse of data will only lead to short-term gain — here we see Access (and Privacy) of ADA (see page 2) come into play. There is no place for those who misuse data, breaking regulations, and they should feel the full force of the law and, perhaps worse, disgruntled consumers who demand brands they can trust.
It’s okay to be opportunistic. If you have a great data asset you can use, or one you can bring in and put to commercial use, then you should do so. The only harm is when this results in data myopia, a short-sighted approach leading to reduced competitive advantage over time. There are a number of ways to make money from your data and none of them are wrong, so long as it serves the consumer and is within the spirit and letter of privacy legislation. Contrasting objectives and propositions can take you down different paths to success. Google, for example, created a search proposition that aided human curiosity for information, then monetised its search engine and is now starting to leverage personal behavioural data to serve targeted recommendations at an increased inventory rate. Google has also augmented its product set and routes to market in order to facilitate deeper levels of engagement with its audience; and all this through advanced access to data.
However, this isn’t the norm as many companies have data and lots of it, but do not know what they should or could be doing with it. Making better use of an existing data asset has long been the desire of marketers, but their challenge has always been, where do I start?
So how do you really plan for data monetisation by design? Acxiom’s five-step model set out in this paper offers a practical roadmap to achieving that goal but first, let’s explore some key areas that will help orientate the marketer/data owner keen to monetise data. The first point at this stage is you should not confuse data strategy with data monetisation. They are closely connected, often overlapping, but not the same. For most organisations the challenge of getting the data explosion in order can seem insurmountable, let alone working out ways to make money from it.
Bringing together a data asset to serve your current business propositions requires a centralised data strategy that serves all business functions and those organisations that have achieved this are held up in the industry as bestin- class database marketers — this is where we see ADA come together, bringing in the Integrity and Availability of the data (see page 2). However, the data strategy itself does not address the challenge of data monetisation; in the context of data monetisation, this could easily be seen as merely the tactical good use of data.
Data Monetisation and Strategic Choice
Most businesses reach a point in their existence where doing the same thing will only result in stagnation; the ceiling where there is no more significant money to be made from existing products, services and markets. At this point you have two choices: become leaner to maintain profitability, or diversify your markets and/ or product base. Diversification is inherently challenging, but strategic monetisation of your data asset can provide you with a very strong revenue opportunity. For some, it may even aid diversification and lead to business transformation.
The diagram on page 6 illustrates Acxiom’s framework for data monetisation beyond existing services.
At this stage, the monetisation strategy is simple: you need to undertand if there is demand for what you know and the data you use to create that knowledge. If there is, you are well on your way to monetising your data. If not, it may be because you do not know your data’s potential or value.
In this scenario, the product dimension is straightforward but your route to market is variable: your distribution and communication channels; the geographies where you operate and, in many cases, a combination of all three. The horizontal and vertical areas closest to these familiar dimensions are areas where more is known about the value of the data. The area between these two thresholds is known as the ‘V zone’.
Organisations are currently looking to monetise their data in two ways:
- Sell data which they use to isolate behaviours and demographics that indicate product take-up or usage
- Sell data used to identify consumers’ preferred communications channels
An example of this might be a telco having very robust telemarketing time-of-day information to build a data product that fuels a non-competitive company’s launch into outbound telemarketing, or taking application data that does not meet your qualification criteria and sell it to partner organisations. Monetisation across the vertical and horizontal axes of the diagram is being successfully tested by a number of companies.
The final target sector (the V zone between the known value threshold) contains the companies that have no resemblance to your own. For a financial services company, this could be a DIY retailer looking to boost local market footfall. Despite the fact that there is no obvious relationship between the two and the financial services organisation would not be able to offer insight to aid the retailer, data on home improvements loans in the local area could fuel a campaign to drive some of that DIY revenue into the store. In reality, neither party knows what data product could serve that initiative, but connecting data from two disconnected companies can amount to a lot more than either could imagine. In that case, the value of the data is whatever the buyer is willing to pay. The challenge for your data champion is to widen your product and route to market knowledge to narrow the V, the zone where you do not know the value of your data. Bear in mind that extending your data monetisation strategy is not advisable until you have a steady revenue stream and can absorb the cost of a less lucrative data product.
If the green area represents your core business, the data and services you know intimately, then your opportunity is to extend out of your core. Analysing the techniques used by extreme mountain climbers, you will observe that they don’t stretch themselves too far at one time. They climb slightly beyond their natural tolerance levels but then return to a more comfortable point to acclimatise and consolidate their efforts before again moving on and up. Taking the concentric circles on the Acxiom framework as our growth waves, by broadening your knowledge beyond your core, the waves become stepping stones to advanced data monetisation. In marketing parlance, this might be considered brand stretch, and the more you stretch beyond your brand, the more opportunities you gain to monetise your data.
For some companies, revenues generated from data could outweigh the contributions of the existing products and services. This tipping point is critical as based on revenue you could argue that you have become a data business. For most organisations, however, data will just become a significant revenue stream.
The Framework and ADA Model — the Chart and the Compass
In many ways, the framework can be thought of as ‘the chart’, where you can plot your business and plan strategies and next steps. Meanwhile, ADA (see page 2) can be thought of as ‘the compass’, the tool you use to validate strategies and plans to practically navigate your business forward in the field of data monetisation.
Planning for Success — 5 Steps to Data Monetisation by Design
Remember ‘The Compass’, ‘The Chart’ and the Consumer
Before the first step and when taking every step thereafter, be guided by the ADA model and use it to navigate across the landscape laid out by the Carter-Devadason Framework. Data monetisation will not and cannot happen without the three essential legs of ADA and while it is possible to be opportunistic generating quick wins, sustainable competitive advantage through superior consumer insight can only be achieved through data monetisation by design, which means knowing where you are on the framework and planning your strategies accordingly. Finally, do not take a single step unless as a business you are aware of the importance of consumer privacy and the essential need for it. In the digital age, quick wins that kill consumer confidence kill brands in timescales of hours and days. Orientate corporate mindsets to serving the customer through data monetisation and the chances of success increase immediately and dramatically.
Step 1: Build your foundation
This might seem obvious but there is a significant gap between what is considered known and what is actually known. If you intend to build data products to make money, then the consumer is king so make sure you understand them. Connect the dots and create a consumer data ‘sandpit’ for your data analysts to work from. The sandpit will also provide insights for your CRM programme:
- Most organisations have pockets of data that are neither known about nor exploited – you need to find them
- Understand and consolidate your existing assets (data synergy)
– This should be at a logical level if not at a physical level. Many organisations work perfectly well with data distributed and indeed within the cloud but all organisations should know what data they have and where it is
– Find the hidden relationships between data items
– Be guided by data governance (privacy and compliance)
Acxiom’s big data principles apply at this stage and the important thing is to know what you are starting with. The IT department will usually know where to find certain data and probably already stores a lot of it in a warehouse; finance departments generate significant, useful business data; commercial routes to market (consumer connection points) have access to a wealth of behavioural data. Meanwhile, data ‘hides’ in customer services, in market research and product surveys; the list goes on. The marketer, who is not always connected with all this data, needs to explore their own business for potentially powerful sources of data. A formal data audit, with the help of a trusted third party, can be highly effective in delivering this holistic and objective view of an organisation’s data and its potential synergies.
Organisations have pockets of data that are neither known nor exploited — you need to find them.
What sort of data should you be looking for?
This grid showing the huge variety of data that combines to deliver multi-dimensional insight serves as a good checklist to begin shaping a data audit. It also supports the Outside-In model (see page 2) when considering the data others may have that could complete your own multi-dimensional data view:
RELATIONSHIP PROFILE
- Customer segment history
- Product ownership history
- Current and lifetime value
- Share of wallet
- Marketing budget by customer
PRODUCT PROPENSITIES
- Propensities across the conversion funnel
- Next best product to sell
- Likelihood to recommend
GEODEMOGRAPHICS
- Age
- Occupation
- Gender
- Location
- Ethnicity
- Family/size of household
- Life stage/life event
INTERACTION HISTORY
- Service history
- Promotion history
- Response history
- Across channels
MEDIA PREFERENCES
- Media consumption
- Explicit preferences
- Implicit preferences
- Context preferences
- Contact/cadence strategy
INTERESTS & ATTITUDES
- Purchase intent by category
- Recreational interests
- Social courses
BRAND ADVOCACY
- Net Promoter Score
- Degree of social influence
- Brand metrics at customer level
BRAND ADVOCACY
- Shopping pathway
- Preferred buying channel
- Preferred research channel
- Preferred service channel
MONETARY INDICATORS
- Income indicator
- Wealth indicator
- Discretionary spending
- Real property indicators
The reality is that the role of data identification is continuous. Your challenge and opportunity is to stay on top of your audience’s interaction and those of potential data monetisation partners. The more you know about the audience, the more value you can ascribe to the data products you create.
Having addressed the issues of finding the data, you then have to consolidate it into an analytical view. How do you do that either physically or at least logically or virtually? There are a number of considerations:
- How large is the dataset?
- Is it structured?
- Can it be connected to a consumer?
- Are the variables clearly explained?
- What consumer permissions are associated with the data?
The marketing strategist, data analyst/statistician and solution developer should work closely as a team to understand how the data should be connected in order to maximise the data product build process. Alternatively, the data scientist or equivalent should take a proactive lead in this area. The analytical view needs to be sufficiently flexible to evaluate the constant flow of additional data coming into the business. A secondary campaign view of the created data products needs to be able to cope with the insight generated from the analytics teams.
There are multiple success points from this stage, however the acid test for all the activities in step one is for the statisticians to find synergies in the data from multiple sources. Ultimately, the combined effect of disparate data should fuel more engaging conversations.
Step 2: Do your homework
Focus on what data and application could together enable the marketer to engage the consumer.
You’ll want to know, what data touchpoint do I need, can I really get at the data I want, create the data products that are fit for purpose and make them available fast enough to be useful at the right touchpoint?
Based on the outcome of the audit you will be able to determine some potential uses of your data. Now you are looking to establish where the demand really lies and how well you can service it.
This is where creativity comes in; finding the right application of data is key. The consumer must be front of mind when thinking about how to better meet their needs, whether that’s through a better product or smarter service, or significantly more intelligent, relevant marketing.
Looking both inside and outside the organisation, what are the unfulfilled needs of the consumer? To be clear, this may be as simple as the belief that they would choose your superior product over another if only you could make them aware of it in the right place and at the right time. So, whether it’s trying to influence major decisions that are made several years apart, like a car purchase; trying to tap into life-stage changes, for example a new house or baby; intermediate purchases like holidays; or which brand of coffee is chosen between the train station and office every day, a creative mindset is required to focus on what data and what application together could enable the marketer to stand out and engage the consumer.
Having developed a deep understanding of your audience, the products they buy and the mechanisms they use to buy the products, you now have the opportunity to monetise data more strategically. The unique consumer view you have, aligned with your expertise, is a highly valuable asset especially to organisations that have synergies with yours. Some synergies are easier to define and price because of their similarity to your core business: these are the ones you should focus on at this stage.
The right approach is to attempt to make it simple for your buyer by using your expertise to create something they can buy: a data or insight product or solution.
Porter’s 5 Forces used alongside Acxiom’s ADA model (see page 2), is a good approach to shaping your product development roadmap. Porter’s dimensions are:
- Market demand
- Potential value
- Competition
- Potential alternatives
- Market saturation
All should be evaluated to determine the attractiveness of a market.
A key benefit to having a structured approach to packaging data for sale is understanding the value your data could have. Your data product could be as simple as a single variable from your database, such as date of birth or an email address, or it could be more complex such as a channel preference model for channel decisioning, a relative income indicator to determine a product price point or online behavioural data to help augment and maximise the value of digital inventory. The important thing is that you as the seller know the value of your data product. Data products should be developed in parallel with a business plan and sales strategy to ensure your data generates a return. If you can articulate the potential value of your asset in this step, then you can better determine how much you can charge for it.
Step 3: Targeted selling
A conversation needs to start somewhere — your responsibility is to balance sales and engagement.
This is the foundational commercial step and therefore ROI is critical. Focus your attention on the opportunities that are likely to generate ‘efficient revenue’. The most attractive data products are ones you can sell. Do this by taking your existing data assets and packaging them for sale.
It can be easy to get caught up in the opportunity, but first, focus on the businesses you know about and where you understand the contribution data can make, and therefore the price you can charge for it.
Basic CRM principles also apply in this step, set out in another Acxiom model, the 5R’s of Marketing.
Recognise your audience, deliver Relevant content at the Right time, be Responsive (keep the conversation going) and nurture engaging Relationships.Then, with the aid of a solid analytics programme and the data, you will drive incremental return. Applying these principles helps align your data products with the consumer levers the buyer can pull; which should enhance buyers’ perception of the value they can realise and reduce the sales time.
This phase can be tactical, offering a solution to the pressure to fix known issues or grab low-hanging fruit.
Interaction data is the primary fuel in this step, telling you what your consumer is doing; all the other data elements help determine the why, how and when. Creating a robust marketing evaluation framework and test-and-learn environment is essential. A conversation needs to start somewhere and you have the responsibility to maintain a balance between sales and engagement. Continuous testing and evaluation will help you hone your tone and message, and in the future will drive your data acquisition and data product strategy.
The opportunity is greatest when you are able to articulate the potential value and application of the data product. This comes with good knowledge of the buyer’s business i.e. an understanding of their market/ proposition or knowledge of the mechanism they are using to engage with their customers.
You also need to make a decision about how quickly the value of your asset will diminish if it is used incorrectly or overused. Privacy conditions will determine access to the data, but governance should protect the data. An additional view of your audience should be created so you cannot just sell your data randomly without recourse. Measures like seeding and usage controls should be taken wherever possible to protect your data from overuse or even misuse. The consumer trusts you to use their data responsibly and preventing data erosion is a key component of any monetisation strategy.
Data governance should not be an afterthought; as previously urged, place privacy and compliance at the heart of your data aspirations. Addressing data privacy capture upstream in a structured way will alleviate potential marketing barriers downstream; ‘easy’ data capture may drive volume, but will not fuel marketing conversations in a sustainable way.
Focused, targeted selling will also stave off potential negativity from internal stakeholders and reinforce your business case going forward. Success at this stage will determine what funding you get for the next tranche of product development and launch.
As a practical example, making data available for digital advertising via addressable advertising provides a low barrier to entry (pushing offline data into the online space). A cookie with behavioural data will have a relatively short lifespan, but offline data will still be available to load into other cookies and can be used in conjunction with multiple behavioural data sources to develop new data products and thus augment the value of your data. Both assets can still be sold separately (on- and offline), but the sales and delivery strategy will vary.
Step 4: New data - think laterally
As your organisational vision expands, existing data might not be enough.
Up to now we have considered what you can do with your existing data assets, but where do you go from there?
Having created a regular and profitable revenue stream from steps 1 to 3 your business ought to be more willing to fund the expansion of the data monetisation programme. To do this you require fresh data. For this reason, collecting data to monetise is a strategic decision. One of the main reasons data is collected is to fuel CRM aspirations. If you can demonstrate a return on your existing monetisation activities, you will have an investment platform.
Ideally, you might be able to create a synergistic data strategy i.e. one that fuels both CRM and monetisation aspirations. This is not a straighforward task as consumer data capture needs a clear value exchange that may differ from the value exchange required to capture data for CRM. Additionally, getting data for monetisation on the capture radar may be at the expense of data for CRM which therefore demands a quantifiable forecasting and evaluation framework.
The ROI should be managed and understood at each stage. Continuously monitoring the business case created prior to all data collection programmes will help rationalise the efficacy of the data, its value and therefore your ongoing data acquisition requirements.
The rapid explosion of data presents both an opportunity and a headache. Planning is key when you are trying to build a data asset that not only fuels your own marketing but also the expansion of your revenue-generating portfolio by taking data products and services to market.
As your business grows and your data fuels a diverse array of organisations you may find that your organisational vision expands. However, your existing data may not be enough to realise that vision.
SELF-REPORTED CONSUMER NEEDS represent some of the most valuable consumer data. The degree of SPECIFICITY and CONSUMER URGENCY are also key factors in driving up the value of the data. RECENCY and TIME SENSITIVITY are major factors because they are an indicator of ‘in market’ status.
Other factors that may also influence the value include AVAILABILITY versus SCARCITY — for example, if dozens of other companies also hold the same consumer insight, the value will inevitably diminish.
At numerous points in your journey, you will identify potential data streams. When evaluating these, you need to ask yourself the following:
- What data are consumers creating (is it relevant and accessible)?
- Is there an application for the data?
- Is there a market appetite for the data?
Consider these questions as your mantra for all planned data acquisition strategies and programmes.
Of course, data partnerships also provide significant growth opportunities in line with the Outside-In model (see page 2). Your organisation’s data combined with third-party data may open up significant revenue opportunities. The same evaluation criteria apply. In recent years, data partnership has become more viable, especially when potentially conflicting data is managed by an independent third party.
Joint ventures also provide rapid growth opportunities. We see this today through the likes of Nectar and Sainsbury’s, Bank of America and a number of high-profile football clubs. Combining expertise to reach new audiences also serves to broaden the range of your competency as well the level of insight derived from your data.
What does your business expansion strategy look like? You may have aspirations to grow beyond your existing portfolio of products and services. A by-product of a data monetisation programme is the knowledge of complementary services you acquire. This knowledge can act as a catalyst for product development and/or mergers and acquisitions. Consider this when determining what you want to collect, where you want to collect it from and with whom you plan to use it.
Step 5: Extend your reach
Steps 3 and 4 are cyclical: the more data you collect, the more data products you can package; and as you broaden your reach, your expanded knowledge of ancillary products and services will continue to fuel new data products to serve completely new industries and customers — just look at how Google has evolved.
At this point you might want to consider what you can do with data you have not already productised. This could be as a result of your organisation not yet understanding the data’s application or value, or you may just have some data captured as a by-product of other data capture programmes. This falls within the V zone of the Carter- Devadason Framework (see page 6). Again, it is important to consider what the market needs and how it wants it packaged.
Alternatively, you could release your data products for general consumption under licence and let the market determine their value. Using this methodology, at little cost, you could publish your unused data into the marketplace as well as your existing data products, then monitor usage and performance to determine a price point going forward. The term “beauty is in the eye of the beholder” rings true here as data that has little value to you may serve another organisation well. This move should be approached cautiously and should only occur when you are comfortable with the sales mechanism and/or you are able to absorb the volatility and associated product development costs.
There are a number of ways of delivering your data to key audiences. Traditional data licensing models are still valid as are the pricing models surrounding them (from CPM > CPR > CPA > Revenue Share) and many companies are developing data management platforms (DMP) to serve their data in real-time across multiple touchpoints. DMPs are becoming increasingly important and cannot be ignored.
Consider what you can do with data you have not already productised.
Conclusion
Data monetisation is related but quite different to the ‘business as usual’ practice of using data to win and manage customer relationships. There’s little doubt those brands that really make data monetisation work stand the best chance of being the winners; brands that not only achieve results but ultimately acquire weaker competitors.
In truth, data monetisation has been taking place for many years; the terminology may be new, but the practice isn’t. What has arrived with the new terminology is an explosion of data caused by the ongoing proliferation of media and consumer touchpoints; however, the increased volume and variety of data sits within brands and organisations, and indeed silos. The ‘magic’ of data monetisation is when potent combinations of data can be identified and brought together to generate significant improvements in consumer insight and marketing results. It is possible to be opportunistic and monetise data tactically, but to be a long-term winner, brands must embrace ‘data monetisation by design’. This means thinking not only tactically and opportunistically, but also strategically.
A practical five-step plan can be followed in line with that vision, guided by the ADA Model (see page 2) and the Carter-Devadason Framework (see page 6) which represent a chart and compass for the journey. The key is where to head next and how to get there; start small, but think strategically. Find the data nuggets that have immediate value. It is better to have one good product than several mediocre products. Remember, step changes aren’t easy, so look to extend your reach one step at a time. And remember the opportunity to monetise data grows when you know your data and your audience. Couple that knowledge with the wants and needs of the organisations most similar to your own business and you can create attractive data products the market will buy.
All of the above advice is crucial, but never forget the consumer. Data monetisation badly conceived or executed will severely wound or even kill brands. Consumers better engaged and served through wellintended and well-managed data monetisation-enabled marketing will buy more and be more loyal going forward. Brands need to make money and it is consumers who have that money. Think of data monetisation as a customer strategy and not as a fast buck.
Data monetisation will continue to evolve as data continues to explode; it will be critical to the success of many sectors and brands, so how committed are you prepared to be? Design your data monetisation for success and you will achieve it.
Want more like this?
Want more like this?
Insight delivered to your inbox
Keep up to date with our free email. Hand picked whitepapers and posts from our blog, as well as exclusive videos and webinar invitations keep our Users one step ahead.
By clicking 'SIGN UP', you agree to our Terms of Use and Privacy Policy
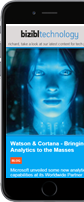
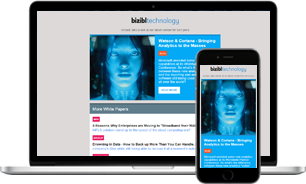
By clicking 'SIGN UP', you agree to our Terms of Use and Privacy Policy