Big Data Big Deal: Five steps to identifying the most valuable 5% of your data
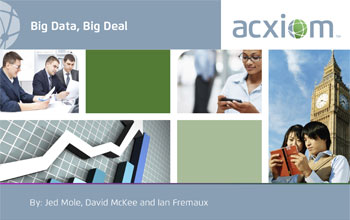
Data has been getting exponentially bigger in terms of rapidly increasing volumes for many years, but Big Data has emerged as a buzzword relatively recently. So how can Big Data help businesses? Download this whitepaper to find out the 5 steps to identifying the most valuable 5% of your data and how you can generate a real competitive advantage from Big Data.
Get the downloadBelow is an excerpt of "Big Data Big Deal: Five steps to identifying the most valuable 5% of your data". To get your free download, and unlimited access to the whole of bizibl.com, simply log in or join free. |
![]() |
Introduction
The premise of this white paper is: accept Big Data is real; but don’t just believe the hype, focus on the desired short- and longterm results and work back from those objectives. It also sets out a five-step plan that will help marketers and brands generate real competitive advantage from Big Data.
What is Big Data? To paraphrase an old advertising adage1 modern marketers could be forgiven for regularly thinking, “I know up to 95% of data is of little value to me; I just don’t know how to find the most valuable 5%.”
Data has been getting exponentially bigger in terms of rapidly increasing volumes for many years, but Big Data has emerged as a buzzword relatively recently. So what’s changed?
When the term Big Data is used today, it generally refers to data that has one or more of three commonly accepted attributes. Some analysts and organisations add others but the accepted three are: Volume, Velocity and Variability.
Volume is the most obvious of the “three Vs” but also the most deceptive. Of course, there is an exponential growth in volume caused by the consumer increasingly emailing, surfing, tweeting, blogging and the like but how much of it is real and interesting? How many of the estimated 4.5 petabytes of videos added online in 2011 (see diagram) were either (a) relevant to the marketer or (b) copies? The fact the individual has copied it may be of interest to the marketer but it’s not new content. Big volume is not new in other fields. The largest single coherent data store in the world is the World Data Centre for Climate at 220 petabytes. It is not volume alone which is changing the world, but volume combined with the other two Vs.
Velocity is a key driver of change in approach brought about by Big Data; the essence of this is that the more interesting behavioural data (think product search) has a limited shelf life and must be acted upon quickly.
Variability of data is unprecedented and a real game changer. Some is transient, some structured and an enormous amount now unstructured yet incredibly powerful. There is a great challenge in taking raw data from the huge variety of possible sources, integrating these into systems and producing actionable insights which feed operational systems.
5 exabytes of data were created between the dawn of civilisation and 2003 — that much information now becomes available every two days.
As Big Data trends ever higher as a topic, it is of concern that many of the recommended solutions involve enormous and urgent investments in IT; the idea being that if you can capture and store all of the data then you stand a chance of generating value from it. The opinion of this white paper is that this is not the answer for all organisations, rather a more efficient solution lies in every marketer asking “so what?” and first understanding how relevant Big Data is to them, how much it can support their business goals and then how to turn potential into reality.
So why should marketers care? The fact is the explosion of data has been caused by the aforementioned changes in consumer behaviour and consumption; the way we shop, work and relax. The growth in numbers and kinds of channels, devices such as Smartphones and all the real-time data they provide through Apps and social networking, products and services has led to Big Data. Consumers have created it, the same people who buy goods and services from the world’s brands. Marketers absolutely must care — this is their space.
Most professionals using data with privacy as a design principle to understand and engage consumers accept a great deal of data is of limited worth. The problem is how to separate the signal from the noise. Somewhere within the avalanche of data are buried significant patterns and behaviours which signpost buying, churn, brand support or aversion. A successful strategy needs to have a mechanism for uncovering these patterns and signals and adapt the operational systems to respond to them.
To achieve the end goal of making their brands more successful by better understanding, targeting, engaging and serving consumers using Big Data, marketers should consider the analogy of human vision which features both ‘focused’ and ‘peripheral’ abilities. We walk down the forest track looking at the path ahead, not seeing every leaf around us, but when we sense movement our eyes immediately turn to the source and respond to the threat or opportunity. Marketers need to create the ability to achieve exactly this, to manage signals but continuously be aware of new signals within the noise and react appropriately.
In the face of much hyperbole around Big Data, marketers and brands first need to avoid panic responses — new trends will always bring big statistics. Instead, think differently about the multidimensional insight Big Data affords. Many businesses have already proven they are excellent at using what consumer insights they have. But could even Apple say it’s driving the maximum possible value from customer data across all its devices and consumer touchpoints? The challenge is going from Big Data potential to the reality of improved business results.
If harnessed properly, all kinds of data can be used to improve a brand’s ability to win, service, keep and grow customers. For example, customer service or product usage data may not traditionally be termed marketing, but in tomorrow’s world, the marketer must want to bring this data to bear having identified its previously unimagined potential value.
Marketing is perfectly placed to make Big Data a business imperative that can serve the entire organisation, not just its own function or the consumer. The modern-day volume, velocity and variability and greater need for validity of data can be managed to make clear the benefits of Big Data far beyond the walls of the marketing department.
The starting point isn’t always clear. However, by implementing the following five-step plan, you will generate quick wins and put the business on a strategic path to better results by making Big Data deliver significant, measurable benefits.
Step 1
Put the Consumer First
Agree the consumer-related business problems you’re trying to address with Big Data
Every marketer knows that for a brand to be successful, it has to have a compelling offer for consumers delivered via the right channel at the right time: the classic Four Ps and the expanded Seven Ps of services marketing. Big Data does not change this, but creates new opportunities. Data can be used to enhance the product, improve the price and make the promotion far more relevant to the place.
One of the challenges is deciding where to begin. Some big organisations have started by loading their largest datasets into emerging technology platforms that solve the volume and variability challenges for their analysts, which allows them to mine these super-size datasets for new insights. Although this is a great way of learning the technology it only provides part of the information you need to define a Big Data roadmap.
The first step of a journey into the fast-moving world of Big Data should be to ensure that you are aligning your efforts to your business objectives.
So, step one is to identify some of the key issues and opportunities that affect your target market today and their relationship with your brand. A brainstorming workshop involving a range of business stakeholders can be used to establish a list of up to 10 priority items to be addressed. This workshop should ensure the business’s strategic goals and key initiatives are considered, taking care to identify which involve the consumer and brand and have the potential to be affected by Big Data. Also, a mixed group of stakeholders will provide ideas for this list from people with different perspectives of your consumers and how you can better align your products and services to their needs.
An estimated incremental revenue or cost saving should be associated with each item on the list. This ROI estimate only needs to be approximate and shouldn’t require an in-depth period of analysis.
At this stage it is not necessary to predict whether the challenges identified can be resolved through a Big Data initiative — that will come later. The tangible deliverable from step one is a list documented in the form of a project brief, with a problem statement describing each item on the list and an impact statement to demonstrate the potential value to the business.
Step 2
Define the Starting Line
Audit the data you have and identify the data you need
In addition to defining the problems and opportunities that Big Data could impact, it is also necessary to have a good view of what Big Data your organisation has. The way to approach this task is by conducting a consumer-centric data audit.
Who should be involved in the process? Outside marketing, IT should definitely play a part as the department is likely to be most at home with data, and may be able to help uncover silos of data unknown to the rest of the team. Data analysts should also be involved as they are already familiar with combining and using disparate data sources and will ask the right questions of the data owners when compiling the data audit. The privacy team should also be engaged to ensure the boundaries of data usage are properly respected.
The audit should create a register of all the touchpoints consumers have with your brand and, for each, should ask questions including:
- What data is generated
- Is it captured anywhere
- Do you have access to it
- Do you have permission to use it
- Does any documentation exist about the data’s structure and content
- Is it structured, semi-structured or unstructured
- Can I join it to any other customer data sources
- Can I get a snapshot of this data for use in a proof of concept?
As recently as five years ago, Acxiom saw an average of 15 to 25 data sources for complex marketing solutions. Now, even mid-tier companies are arriving with 50 to 100 data silos which require integration.
Since the advent of social media it is even possible to start capturing information about what your consumers think of your indirect marketing campaigns.
The next step is to collate more detailed information about the sources you can access. Hopefully for some of these you’ll have lots of information, but others may be reliant upon key expert users, and some will be relatively unknown. At this stage you should aim to obtain a profile of the main data entities and attributes from each system — the ‘data dictionary’ — which includes the spread of values and their frequencies for the attributes. Software tools can be used to automate some of this work. This process will highlight where data generated at a consumer touchpoint is unavailable. Filling in these data gaps is something that could be included in the strategic roadmap later in the process at step five.
Each data source should be scored on a number of measures that can be used to assess whether it is a valuable source for inclusion in a proof of concept. For example, your web analytics data might score high on granularity of information available but low on completeness if you can only access the last few days’ worth.
The final thing to include is data sources that you would need to have a better understanding about your customers and prospects.
The specific deliverable from step two is production of a comprehensive list of the raw materials you have available for any Big Data initiatives and identification of the gaps where the data is unavailable.
Step 3
Create ‘Plan A’ — A Proportionate Response
Plan to test and invest, proportional to the strength of your analysis and findings
Having understood the objectives and the current landscape in steps one and two you must now build a strategy for managing and making Big Data actionable — a ‘Plan A’. The challenges here will be familiar if you’ve ‘sweated blood’ building your corporate data warehouse, but are magnified in the context of Big Data by the volumes and variety of data involved. Furthermore, the velocity factor means that unless the data gets processed and actioned rapidly then the data quickly becomes stale.
Throwing all of the data into a new platform like a large Hadoop cluster is not really going to solve the problem in isolation. Yes, you are going to need some hardware and processing power, but a strategy for doing something useful with raw data is critical. Enlisting the skills of an entrepreneurial senior analyst at this stage could prove vital.
Solutions will vary from brand to brand but any Big Data plan must address how to:
- rapidly on-board new data sources
- analyse raw data to determine when and how it can be used
- make data operational quickly and efficiently
- identify, and if necessary discard, junk data which will clog the system
- automate decisioning to accommodate the variety and velocity of Big Data
- ensure all activity is executed in a privacy-compliant manner.
Consider the enormous volumes of website tracking information generated by a large consumer site. Some facts will be useful, if only for a short time. For example, a recommendation engine may take a real-time feed of product searches to determine which adverts to display to users; an abandoned basket is a trigger for retargeting. The problem is much of this data is just noise. However, data mining can identify patterns or activities which are predictive of some desired behaviour and once the pattern is identified the data can be actioned by using it to feed the campaign or decision system.
It is the agility in the face of an everchanging data landscape, and not just volumes, which distinguishes Big Data solutions from previous incarnations.
By bringing together the learnings from steps 1 and 2 with an appreciation of the possibilities offered by the new technologies, the specific deliverable from this step should be a practical, initial vision and plan of how Big Data can work for you and your organisation.
Traditionally, this is the beginning of the tender or RFP stage where firm decisions are made about hardware, software and service providers. However, given the scale of investment, and to ensure ‘the Emperor really is wearing some clothes’, the next step is a Big Data reality check.
Step 4
Test Big Data
Deploy a proof of concept to test and learn
Clearly there may be a significant investment required to establish a Big Data environment, not just in terms of hardware but also in terms of skills. Demonstrating ROI is therefore mandatory in securing sponsorship from the business.
One of the paradoxes of Big Data is that many of the use cases do not actually require Big Data. The volumes are so large that the decisioning necessarily becomes simplified. With the exception of some parts of the automated decisioning aspect, there is nothing fundamentally new about Big Data. It’s just a new mindset that’s required.
What this means is that it should be perfectly possible to conceive of and execute Big Data use cases without building a full-blown Big Data environment — especially for a proof of concept.
It’s fair to say that if you can’t demonstrate a handful of use cases from a marketing viewpoint, then it’s unlikely that any Big Data environment will pay its way. Consider the SETI@home project (see below). It was necessary to prove the model worked first with a small number of networked computers in a lab before upscaling to cover 3 million computers worldwide.
The resources and effort required to prove a use case will vary. In some cases smaller volumes of static data may suffice, in others production-scale volume may be required. A partner with an existing large-scale analysis environment may be able to help you look for the early wins which will justify the business spend. The objectives of step four include:
- establishing which data sources offer useful, predictive information. This can be done with static physical dumps of likely sources (sales, web-logs, call centre etc)
- establishing which external sources are required and may need to be brought into the mix (socio- or geo-demographic data)
- providing metrics for any predicted uplift, based on extrapolation from the proof of concept
- manually executing the integration steps — which must be automated in live environments — so they are understood and conform to privacy regulations.
Typically, the activities at this stage will include statistical analysis (mining), searching for predictive patterns within the data and then attempting to turn these into processes which can be tested in real-world scenarios. Once again, this is where third parties can help. A good external partner should be able to provide resources such as data scientists and technologists with a grasp of the new software and platforms, to fast-track learning at this stage.
Moving forward from this step, the specific deliverable should be a solid body of evidence to support the business case, and a clear understanding of the resources and processes required to underpin it. You ought to be in a position to present ROI cases to leadership, and to begin validating and adapting ‘Plan A’ from step 3, producing a strategy for Big Data that will be delivered through a practical roadmap.
SETI@home was the second large-scale distributed computer project set up in the 1990s (Berkeley University & US Space Labs). It had the following objectives:
- to do useful scientific work by supporting an observational analysis to detect intelligent life outside Earth, and
- to prove the viability and practicality of the ‘volunteer computing’ concept and conform to privacy regulations.
Whilst the first objective has not yet been met the second was an almost complete success — demonstrating how home computers could be used to process huge amounts of data in a collaborative environment. SETI is one of the fathers of cloud computing.
Step 5
Emulate Human Vision — Focused and Peripheral
Create a roadmap to operationalise proven approaches, and identify and test new ones
Finally, you are ready to build on the results of the proof of concept. As previously described, the ideal state is to achieve both focused and peripheral vision: the ability to focus on the data that matters most while being aware of other data, and be constantly on the lookout for new or previously unavailable data.
Step five is about creating a strategic roadmap. This will outline how any proof of concept tests can be operationalised, part of ‘business as usual’ and how the organisation will move forward with subsequent proofs of concept already defined. It will also implement your peripheral vision.
Steps three and four will hopefully have armed you with information to build the business case to move forward; an incremental return on marketing investment with well-managed risk should have the business’s budget holders happy to ask: what’s next?
The effort required to make progress should not be underestimated but likewise needn’t be a cause for alarm. While Big Data can be ephemeral and difficult to tackle head-on and while the business will need to invest to make Big Data proof of concepts operational, the fact remains that the majority of what the marketer is trying to achieve, is getting the right data in the right place at the right time.
The quickest, and arguably best, return will almost certainly come from a marketer’s ability to bring high-value data to bear in existing marketing systems and programmes.
To this end, priority consideration should be given to what Big Data can most quickly be captured and translated into these existing environments. You need to conduct a value analysis and prioritise. For example, compare the investment in the capability to take unstructured data and turn it into large volumes of structured insight against the expenditure needed to capture a more modest volume of structured data. The yield of each option must be identified and the answer may be to do both. It is worth remembering that much of this capability can be implemented between the consumer-generated Big Data and the existing systems without the need to completely rebuild existing architectures.
It should be clear by this stage that the five-step approach offered is one that allows businesses to avoid a crippling ‘knee-jerk’ investment in reaction to the opportunity and threat Big Data represents. A proportional response that takes practical, informed steps to generate significant results is vital. Once you have a clear roadmap to operationalise the initial proofs of concept and deploy the next batch - the focused vision - attention needs to be given to how the marketer achieves peripheral vision.
The good news is that most of the activities undertaken in steps one to four contain much of what the marketer needs to deliver peripheral vision. You must put in place a programme of continuous activity, indeed for many organisations this should entail a separate team that specialises in scrutinising the evolving Big Data universe to identify and evaluate potentially powerful sources of Big Data. This team is like weather forecasters, always taking readings and measurements, using a range of permanent and ad hoc activities and tools to predict what’s coming next.
The very nature of Big Data means the roadmap for organisational change should always be based on testing and refining. This team needs to consider the potential of the data from an analytical perspective but must also apply pragmatism around the challenge they are going to present to their colleagues tasked with trying to operationalise the data. They should also be able to assess the likely impact from a marketing systems point of view and be capable of framing their findings and recommendations in terms of return on marketing investment.
The key deliverable from the final step is a strategic and tactical plan, including a roadmap that operationalises Big Data trials, generates results, enables learnings and identifies potential opportunities so that marketing, the brand and the consumer continuously benefit from the exploitation of Big Data.
Conclusion
If Big Data was already present in the past, albeit with its own fast-growing volumes, it was based on what now seem narrow variables such as location, age and salary. These days, the layers and levels are almost limitless; where are people located when they use HTML5 mobile applications, how many brands do they purchase from and through which channels, what do people think and how do they feel?
Big Data is real and represents both an opportunity and a threat. The threat comes in the form of putting prime focus on getting and hosting as much data as possible without regard to how it can be most profitably used. Like the donkey chasing the carrot that’s always out of reach, the pursuit of being able to catch all the data available as it evolves is always likely to result in disappointment.
The opportunity becomes more realistic and tangible once a business shifts from trying to solve all of its problems with all of the data available. Opportunity comes from focusing on what problems Big Data stands the best chance of solving, understanding where the organisation is today, creating a ‘Plan A’, testing and then building a roadmap that gives the marketer both the focused and peripheral vision to make Big Data deliver incremental return on marketing investment.
Given consumers create Big Data and brands need to engage, serve and delight them to be successful, it follows that marketers are best-placed to plot the course towards finding that golden 5% of your Big Data, ensuring it delivers benefits to both the consumers and the brand.
Big Data has been evolving and growing in the background for some time. Now it’s here, can you afford not to take it on? Just make sure you take it on in the right way and on your terms.
Acxiom and Big Data
Acxiom can help marketers achieve results by exploiting the potential of Big Data with deep understanding of how to identify the right data and put it to profitable use. Combining marketing and consumer expertise, technology and data, Acxiom provides solutions that are fully compliant with privacy regulations and support the five-step plan, grounded in a ‘proportional response’ approach, and can evidence the benefits with case studies that showcase more than 40 years of data expertise.
Contact details
For more information call 020 7526 5265.
www.acxiom.co.uk
ukenquiries@acxiom.com
Want more like this?
Want more like this?
Insight delivered to your inbox
Keep up to date with our free email. Hand picked whitepapers and posts from our blog, as well as exclusive videos and webinar invitations keep our Users one step ahead.
By clicking 'SIGN UP', you agree to our Terms of Use and Privacy Policy
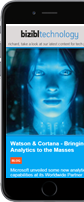
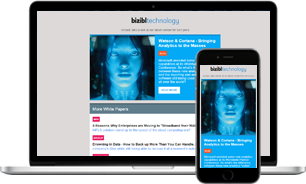
By clicking 'SIGN UP', you agree to our Terms of Use and Privacy Policy